A Model for Predicting Where Landlords May Be Skimping on Heat
October 8, 2015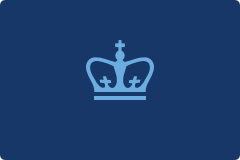
Top Hackathon Prize Goes to Project Analyzing NYC Heat-Complaints
Thousands of New Yorkers complain each winter that their apartments are too cold. To make it easier to verify complaints and get relief, a group of student hackers developed a low-cost temperature sensor system that sends hourly updates to their servers at Heat Seek NYC.
A new feature, developed this past weekend at the Columbia Data Science Student Challenge, goes a step further by predicting where heat complaints are most likely to occur, potentially helping city officials prioritize investigations. Called GotHeat, the project was awarded the top prize at Columbia’s hackathon, hosted by the Data Science Institute and sponsored by Microsoft. The developers, Daniel Kronovet, Roberto Martin and Sam Guleff, each received a Microsoft Surface Pro tablet.
The team began by filtering the million or so heating complaints lodged with NYC’s 311 hotline over the last five years. The complaints originated from just 100,000 buildings, they discovered, many of them rent-stabilized and cross-listed in a separate database that included the building’s age, size and other attributes. They narrowed their dataset to these 43,000 rent-stabilized buildings to explore additional risk factors.
Separately, they developed a proxy for risk based on the number of apartments, total complaints and pattern of complaints over time. The attributes of buildings that scored in the top 40 percent were incorporated into their model as a generalized risk factor. Experimenting with other predictive features, they settled on ZIP code, building age and number of apartments. With those three inputs, their model infers whether any building in NYC is at risk for heat complaints.
The 16 teams that competed over the weekend had 24 hours to come up with a working model. Most of GotHeat’s time was spent cleaning, joining and aggregating the data. “When we were ready to learn our classifier, [Microsoft’s machine learning service] Azure made it relatively easy to build, evaluate and deploy the model,” said Martin, a software engineer at Bloomberg studying for a master’s in Data Science at Columbia.
At 1 am on Sunday, the team decided to shift from trying to improve their model’s accuracy (then at 77 percent) to building a user platform. “It’s more product management than data science, but I think we won points with the crowd by having a working prototype of our tool,” said Kronovet, who is a master’s student in Columbia’s Quantitative Methods in the Social Sciences program.
Kronovet helped develop Heat Seek last spring as a student at the Flatiron School, a coding academy in Manhattan. He and his friends had read about landlords harassing tenants by withholding heat and wanted to help. (Building owners are required by law to keep temperatures above 68 degrees Fahrenheit during the day and above 55 at night). With a $30 networked temperature sensor and a $60 hub, they figured out a way for tenants to record and report heating violations.
Last fall, Heat Seek won $25,000 in prizes at the fifth-annual NYCBigApps competition. Now a registered non-profit, Heat Seek has a full-time CEO and a volunteer staff of seven.
As chairman of Heat Seek’s board, Kronovet hopes to polish GotHeat and incorporate it into the system. He wants to put his team’s dataset of 43,000 buildings—including the 17,000 or so deemed high-risk for heat complaints—online. He has also contacted the office that investigates heat complaints, NYC Housing Preservation & Development, about adapting the model for city use.
Other Data Science Challenge finalists:
A team of four women–Yubo Han, Yiqian Jin, Yue Guo and Ziyan Feng–won first place and $3,000 for their project, “Help4,” which predicts how long it will take NYC’s Office of Emergency Management to close a case based on the date, time of day and weather when an emergency call is received as well as the type of incident.
Second place and $2,000 went to Gary Sztajnman, Robert Dadashi-Tazehozi, Brett Averso and Aleksandr Makarov for their project, “Ratspector,” which looked at NYC restaurant complaints and complaints about water quality, garbage and rats, to predict where rats are most likely to be found.
Third place and $1,000 went to Carolyn Morris, Pedro Perez and Woojin Kim for their project, “Visualizr,” which predicts the borough someone lives in based on his or her age, gender, ethnicity, income and commute time to work.
— Kim Martineau