Andrew Miller Formulates New Algorithms and Machine-Learning Models to Improve Medical Science
August 31, 2018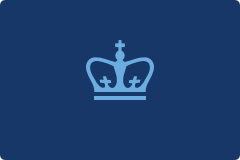
When he was a boy growing up in New Canaan, Conn., Andrew Miller was compelled by his well-meaning parents to take piano lessons. He loathed the lessons, though, and as soon he was old enough to negotiate with his parents – at age 13 – he switched to guitar, which he happily played in high school. He liked sports, too – he ran for his school’s cross country team – and when he wasn’t watching NBA games he could be found playing pickup games of basketball. Academically, his favorite subjects in high school were math and physics.
In college at Brown, he majored in computer science and music, where he focused on composing classical music infused with jazz for which those boyhood compulsory piano lessons served him well.
“I definitely think there’s a tie between music, computer science and math,” says Miller, “especially being able to abstract certain concepts or nuggets and repurpose them in other contexts to create something new.”
For his senior thesis at Brown, he composed a modern composition for a piano quartet and a percussionist. And he wrote a jazz-influenced piece for a saxophone trio. What he likes best about composing and playing in small combos is the collaborative nature of improvisation – the same quality he likes best about data science.
“With improvisation, you’re trying to collectively create something totally new,” says Miller, who recently earned a doctorate in computer science from Harvard. “Similarly, with respect to data science, what I like most is working with experts from a totally different field, and coming together to solve a problem in a new way.”
In his postdoctoral research at DSI, Miller will allow for new collaborations as he attempts to formulate new algorithms, statistical methods, and machine-learning models that can be used to improve aspects of medical science. While he hasn’t formulated his postdoctoral project yet – his postdoc begins in September and he’s consulting with his advisers, Professors Dave Blei and John Cunningham, to refine his project – he knows the focus will be on using data science in medical research.
Among the work he’s considering is a project he started at Harvard applying novel data techniques to electrocardiograms to monitor the heart. Miller would like to refine the screening procedure so it can potentially predict health complications, such as future heart attacks or atrial fibrillation. Electrocardiograms, or EKGs, can predict certain cardiac conditions such as arrhythmia. “But the goal of this project,” says Miller, “would be to detect these kind of pathologies at earlier stages. Are there subtle patterns in ostensibly normal cardiac function that give us information about future arrhythmias or heart attacks in, say, 72 hours?”
The question he will try to answer in his research is: What information is contained in raw EKG signals that could be analyzed differently to refine doctors’ measurement of cardiac function? There are new machine-learning methods that have been applied to EKGs, but the field is developing. He hopes to merge deep learning with statistical modeling to create a prediction algorithm that could offer probabilities on whether a patient will have a heart attack or exhibit an atrial fibrillation in, for instance, the next three days. In analyzing large numbers of EKGs, the data could help detect variations in the signals and lead to better understanding of how they correspond to cardiac functions in thousands of patients.
“These deep-learning algorithms are new, and most doctors wouldn’t necessarily be aware of them,” says Miller. “But if successful they can be immensely helpful in enhancing doctors’ ability to detect heart conditions in patients before they escalate.”
Miller is also considering a project in which he’ll apply machine-learning algorithms to collections of electronic health records (EHR). The patient data he collects come in the form of various tables detailing lab tests, vital statistics, diagnoses, medications, and past medical procedures. The data include information on patient visits, medications, vital signs, and observations such as electrocardiogram tracings, echocardiography or ultrasound imagery. He’ll combine these data to better characterize a patient’s overall health.
But EHR data can be messy, often containing missing entries (e.g., unobserved treatments), sampling bias (e.g., physicians selectively issuing lab tests), and high-dimensional observations (e.g., electrocardiogram tracings and echocardiogram images).
“These complexities present a huge statistical challenge that must be addressed with the development of new techniques,” Miller says. “I hope my research can help solve some of these challenges, so that doctors and scientists can predict and understand potential health problems in patients and intervene to help them.”
As a DSI postdoctoral fellow, he’s excited about partnering with researchers from every corner of Columbia University.
“What I enjoy most about data science is its inherent interdisciplinary and collaborative focus,” he says. “DSI researchers work jointly with people from across Columbia and all over the world, which is why I’m excited to be a part of the inaugural class of postdoctoral fellows at DSI.”
— Robert Florida