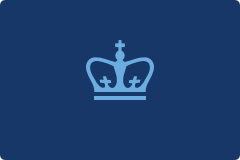
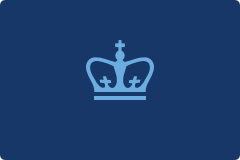
In their final semester of the master’s program, DSI students take a Capstone class, where they divide into teams and work on data-science projects that give them invaluable hands-on experience in the field.
The teams work under the direction of industry affiliates, who guide them through the entire cycle of how to use data science to solve real-world problems. The DSI affiliates, a mix of corporations, government entities and non-profit agencies, help the teams define their projects, establish their goals and set deadlines. Sometimes a Capstone project will prove so successful that the affiliate will use the team’s findings to help improve data analytics at their company or organization. The students work on the projects for the entire semester, at the end of which they present their findings to their professors, affiliates and fellow students. And given the number of talented students at DSI, it’s no surprise that the final projects are impressive.
“I was delighted with the results of their projects and know that this experience will serve them well when they graduate from DSI,” said DSI Professor Andreas Mueller, who taught one of the Capstone classes.
For their part, the students say they gain experience in demonstrating and sharpening their data-science skills – experience that serves them well when it comes time for them to interview for jobs. As it is, students who study data science are in high demand, but acquiring hand-on experience enhances their marketability, since they can discuss their projects with recruiters.
“Working on the Capstone project gave us a complete understanding of the responsibilities of a data scientist,” said Sanjmeet Abrol, who belonged to a team in Professor Eleni Drinea’s class that built a recommendation system for Instacart, the online grocery firm. The system had two intents: to recommend products to customers and to create a predictive tool for Instacart that shows when the customer orders would arrive and what products would be in the orders.
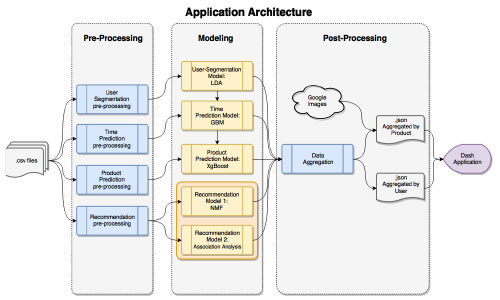
The team used data from The Instacart Online Grocery Shopping Dataset 2017, which contained a sample of more than three million orders from 200,000 Instacart users. They found that products from the fresh fruits and fresh vegetables aisles appeared most frequently in orders, and that bananas were the most popular product. They also observed that organic products were highly popular among Instacart customers.
The team’s findings will help Instacart build a user-product recommendation system and provide customer segmentation that will help the company plan marketing campaigns.
Team: Sanjmeet Abrol, Alexander Castleton, Kaavya Chinniah, Tarek Elelimy, Gregory Johnsen
Industry affiliate: Synergic Partners
Using Data Science to Help Police Combat Crime
Can data scientists use crime statistics to unearth patterns that will help law enforcement officials reduce crime in New York City? Could the crime data, moreover, be used to predict where and when crimes are mostly likely to occur?
For their project, this team sought to answer these questions by compiling statistics from New York City’s Police Department’s Historic complaint data, which includes felonies, misdemeanors, and violations reported to police from 2006 to 2016.
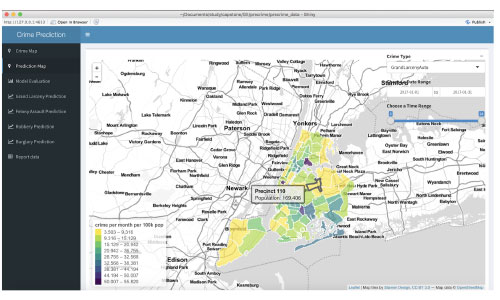
The team focused on seven felonies: homicide, rape, robbery, burglary, grand larceny, grand larceny auto and felonious assault as well as six offences: weapon possession, arson, fraud, forgery, drug offenses and criminal mischief. It was a large dataset – nearly two million felonies – and to make it more manageable the team created an interactive crime map of New York City. The map shows the occurrence of crime for the 10 years (2006-2016) covered in the police data. They also designed an interface so that users could retrieve data on the type of crime, the location, and the time it occured.
They were not able to predict where crime might occur but their crime map, along with their interface, could help police gain an historical understanding of crime in the city. And police could then use that understanding to decide where to deploy anti-crime units. Citizens could also use the team’s system to see where crimes are mostly likely to occur and what neighborhoods have the most crime.
Team: Panpan Cheng, Vibhuti Mahajan, Franck Ngamkan, Jared Samet
Industry Affiliate: Synergic Partners
Impact of Amazon Warehouses on Surrounding Areas
This team partnered with Capital One to study the impact Amazon facilities have on surrounding areas. The group analyzed two datasets: The first was publicly available data on the location of Amazon warehouses, and the other was economic and demographic indicators from the U.S. Census Bureau.
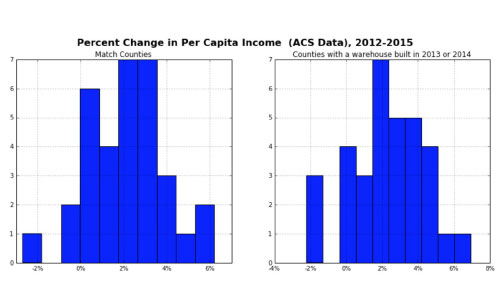
Using specific indicators such as population, income and age of residents for a three-year period (2012 and 2015), the team compared how counties with Amazon warehouses differed from counties without Amazon warehouses. They found that the presence of the warehouses had little to no effect on counties.
Counties without an Amazon warehouse progressed in a similar way economically and demographically as those counties without a warehouse. Additionally, they found it’s possible to create a predictive model that could determine where Amazon was likely to open a new warehouse – a matter very much in the news these days.
Team members: Alex Wainger, Sam Somuah, Jessica Raab and Qiong Hu
Industry affiliate: Capital One
Estimating demand for taxis at airports
This team evaluated the supply and demand of taxi service at LaGuardia Airport, and what they learned gave them pause: most taxis end up waiting three hours at the airport to pick up a passenger. The long wait makes drivers reluctant to come to the airport, which in turn makes it even harder (and longer) for passengers to get a cab.
Using data on incoming flights, weather patterns and departure times, the team trained a neural network to predict the demand for taxi pickups at LaGuardia Airport. And the bigger question the team addressed was this: In terms of the passengers arriving at the airport, what percentage choose a taxi over other modes of transportation? Based on input from their partners at the Taxi and Limousine Commission, the team estimated that 15 to 20 percent of passengers arriving on flights at the airport choose taxis as their mode of transpor
The team’s model can be used by the Taxi and Limousine Commission to accurately predict taxi pickup demand at LaGuardia Airport, thereby improving service for both drivers and passengers. The model can also be used as a case study to justify better data-sharing practices between the Taxi and Limousine Commission and the Port Authority.
Team: Adam Coviensky, Anuj Katiyal, Keerti Agrawal and Will Geary
Adviser: NYC Taxi and Limousine Commission
Understanding water quality and composition in South Africa
This team partnered with Unilever, a consumer goods company, in identifying datasets that characterize the composition of water in South Africa, a high-business region for the company.
The aim of the project was to create a dashboard showing water-monitoring stations and metrics for pH, hardness of water and electrical conductivity. The team analyzed datasets from the Department of Water and Sanitation of South Africa. The group also evaluated data from Gemstat, a dataset with details on water quality at both global and local levels. They found that the department has 3,385 water-quality monitoring stations positioned throughout the country and that the stations monitor concentration levels of 16 elements found in the waterways.
The successful project is still continuing and the team is working with Unilever on how it can best use the dashboard to get clean water for use in the production of its products.
Team: Conrad De Peuter, Baran Akyol, Haiqi Zhu and Xikai Chen
Industry Affiliate: Unilever
Analyzing H1-B Visas and the Demand for Foreign Workers
This team studied how the strength of the American economy affects the demand for H1-B visas. The visa program enables American companies to temporarily employ foreign workers who have specialised skills, resulting in large number of applications each year.
To analyze the fluctuation demand, the team used datasets from Labor Certification Applications for the years 2001 to 2017. They also developed time-series models that predicted the number of applications filed for three years, and indicated which industries filed them.
The team found that visa caps and the availability of alternative visa classifications affected the demand for H1-B visas. The Optional Practical Training (OPT) extension for STEM degrees, for instance, gives much flexibility to employers and job seekers, thereby altering the demand scale in the H1-B visa category.
They also determined that there was a dip in the visa demand due to recession in the years 2009-2012. In 2009, moreover, there was a drop in the number of workers requested due to a new system of visa caps.
The team successfully evaluated the demand for H1-B visas and even created an interactive application with visualizations to illustrate how the demand for the visas fluctuates.
Team: Pawel Buczak, Tejas Dharamsi, Daryl Kang, Hung Shi Lin and Marika Lohmus
Industry Affiliate: Goldman Sachs
Passenger behavioral pattern in Madrid’s Metro
In this project, the team profiled passenger behaviors in Madrid’s underground metro stations and studied the commuting patterns of metro riders in different stations.
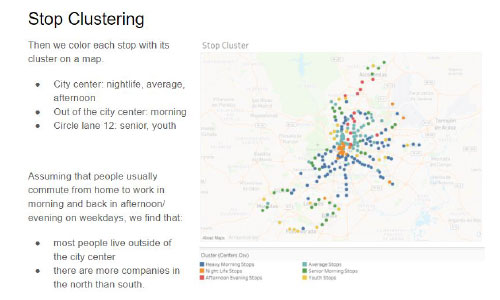
For their main dataset, they used the metro ticket validation for May 2016. One challenge the team faced was the absence of exit-data from passengers, which would have help them to discern commuting patterns. Additionally, it was difficult to identify transfer passengers without their destination data, and these passengers often constituted a group with interesting characteristics. The team, however, came up with solutions for this missing data by using turnstile data to supplement ticket-validation data.
The team performed segmentation analyses to understand how passengers select and prefer certain stations. The final outcome for them was clustering behavioral patterns in the form of maps and charts. The team believes that its work can help metro riders better plan their trips as well as help marketing companies to target specific audiences.
Team: Hanlin Wu, Rachel Zhang, Tianxiao Ye and Chang Pan
Industry affiliate: Synergic Partners
Using Deep Learning in Ad Conversion Prediction
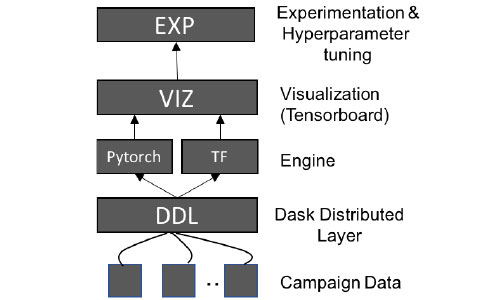
Online marketing companies use machine-learning models to predict which ad campaigns will be clicked on by users and they often use those predictions to target prospective customers. In this project, the team used data provided by MediaMath, an online advertising company that targets millions of internet users, and used deep-learning and other techniques for their analysis
The team used data from nine different marketing campaigns of MediaMath. Each data point was associated with a label indicating if the ad was clicked (‘1’) by the user, or not (’0’). The team also highlighted and categorized the important fields relevant to the model. An advertiser could use their analysis to indicate if a user had been on its site.
In terms of modeling strategies, the team focused on train-validation-split of the data. The team plans to further partner with their affiliate on their findings and modeling strategies. Moreover, they want to explore other models that can potentially run faster yet provide similar results.
Team: Aarshay Jain, Abhay Pawar, Aravind Sadagopan and Karl Loic Kamdem, Vinayak Bakshi
Industry Affiliate: MediaMath
Overall, the Capstone students said they learned a great deal from their projects, which taught them the importance of teamwork; how to communicate results to different audiences; and how to divide work and meet deadlines.
“The Capstone project gave us a chance to implement everything we had learnt in our classes and gave us the experience we needed to confidently enter the workforce as data scientists,” said Abrol, the student who worked on the Instacart project.
What follows are brief summaries of the projects done by students in Professor Andreas Mueller’s Capstone class.
Designing a Search Engine to Help Home Buyers Find the Right House
The team designed a search engine that assists home buyers in Madrid by providing them with data on housing options as well as on neighborhoods, schools, hospitals, local amenities and more. Their search engine then matches a buyer’s preferences for housing and neighborhoods with a list of available houses or apartments.
Most search engines in the field offer limited information to buyers since they don’t provide information on a neighborhood’s schools, socioeconomic status, or services. The team thus created a search engine that offers prospective buyers data on both housing features and neighborhood attributes.
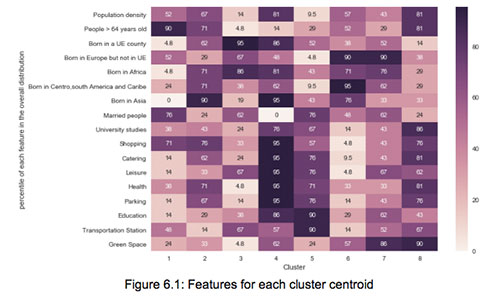
For their data, they scraped listing-datasets from Idealista API, a leading real estate portal in Spain; used demographic data from the Spanish National Institute of Statistics dataset; and compiled data from OpenStreetMap, a nonprofit that provides free geospatial data.
They used an algorithm (K-Means) to cluster the 21 districts of Madrid into homogenous groups. Houses were recommended to users by identifying the cluster that matched a prospective buyer’s input preferences. The engine also recommended houses in the cluster nearest in proximity to the buyer.
The team was satisfied with its results, but hope to further enhance their recommendation engine by incorporating more data sources. The distance of each house to important town services such as schools could be valuable in making and ranking house recommendations. And if they had data on individual buyer behavior, they could use that to also enhance their model.
Team: Qi Chen, Xiulin Hou, Wenyue Li, Qing Ma and Kevin Ma
Industry Affiliate: Synergic Partners
Using Data Science in Pro Soccer
Though soccer is the world’s most popular sport, data analytics is used more sparingly in soccer than in other professional sports. This team used data science to analyze an aspect of soccer that could immensely benefit coaches, agents and managers.
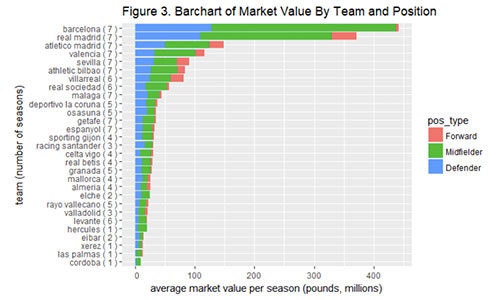
They used data science to identify soccer players in La Liga, the elite Spanish league, who are over-performing relative to their market value. If coaches and managers could definitively identify players whose performance exceeds their market value, they could improve their team’s performance by signing good players relatively cheaply.
To develop such a model, the team analyzed seven years of data about La Liga players. They merged player-level performance data from WhoScored.com with metrics, players and market values from Transfermarkt.
They then predicted a player’s market value by using linear regression and Random Forest models, a method for classification, regression and other tasks. The model predicted the market value of players based on an array of performance indicators. It also compared a player’s market value to his salary and, most importantly, determined if he is undervalued in the league, an assessment that would benefit coaches, agents and managers.
In the world of soccer, where chance and skill are equally present, the team hopes its model can be used as a tool to provide coaches with an increased level of certainty in evaluating players and creating better teams.
Team members; Chris Halpert, Molly Hanson, Rohan Pitre and Feng Ye
Industry affiliate: Synergic Partners
Does Age, Body-Mass Index and Smoking Affect Genes?
In their project, the team studied the interactions between gene methylation as it relates to three factors: a person’s age, body mass index (BMI) and smoking habits.
Gene methylation is an mechanism used by cells to control gene expression. But how is methylation affected by age, BMI and smoking habit? The team used recently released skin aging datasets on 32 subjects from Multiple Tissue Human Expression Resource methylation data, a part of the Gene Expression Omnibus dataset from the National Center for Biotechnology. That data included information on age, BMI and smoking.
DNA methylation occurs when a methyl (CH3) group is added to a DNA region known as a CpG site. Studies show that methylation can be affected by both environmental and genetic factors. One study found that methylation is typical in aging cells; another study on BMI identified associations with health; while a study on smoking found 66 differentially methylated CpG sites. All three studies have identified changes in methylation at several CpG sites associated with age, BMI, and smoking status.
Their dataset was collected from skin samples and their models were built while considering other predictive variables. They searched the available literature and found that the same CpG sites were related to smoking in other kinds of tissues. And using the methylation data from skin tissue, the team successfully found a list of CpG sites that are significantly linked to age, BMI and smoking.
Team members: Aditya Garg, Chenchao Zang, Jun Guo and Papiya Sen
Industry Affiliate: Unilever
Helping Merchants in Madrid Find the Best Location for Businesses
Most people have heard the mantra: location, location, location. If a small business is to exceed, the most important factor is finding the right location. And to help prospective merchants in Madrid do precisely that, this team created a website that provides data on credit-card transactions as well as on demographics. Prospective merchants can use that data to analyze possible locations and to see how different kinds of merchant performed in those areas.
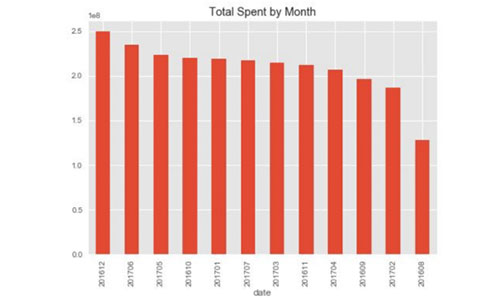
The team also used a public dataset of banking transactions processed by the Banco Bilbao Vizcaya Argentaria (BBVA), a Spanish bank, to understand consumer behaviour in the different locations, information that is also valuable to merchants. From this, they also designed an interactive map with different layers that visualize the above data and give an overview of the consumer landscape in Madrid. Merchants can use the team’s customized system to see how different businesses performed in different locations – essential data to consider before choosing a location.
Team members: Brett Averso , Matthew Dawidowicz, Kevin Ma and Mark Salama
Industry Affiliate: Synergic Partners
Unilever Body Wash Project
This team studied Unilever’s body-wash products, especially in terms of their lathering and foaming properties.
They evaluated three datasets (customer surveys and internal expert panel data on products) provided by Unilever to understand the customer’s perception of body wash products, especially their foaming and lathering properties. Their premise was that body wash lather is a key factor in how consumers perceive Univlier’s body-wash products. And it’s also important to tailor the lather experience of products to meet customer preferences in different markets. While customer perception of body wash lather is understood through survey data, the production of body wash is based on laboratory-sensor measurements. Regarding the latter, the team found that there was a lack of understanding of what lab measurements are beneficial in creating a body wash product with appealing lather properties.
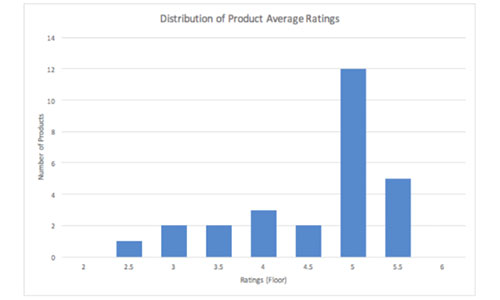
They found there is a stronger relationship between technical lab data and sensory profiles. They therefore proposed a robust list of features to describe the chemical process by computing different metrics on a time series generated by the foaming machines. Some of these derived metrics were significant when regression was applied on sensory profiles and technical data.
The team’s research is a step forward in helping Unilever better understand how customers respond to its products. Their study could help Unilever replace expert panels with lab measurements to some degree, and to see which lab measurements are most helpful in predicting how lather is perceived by customers. They hope their study will help Unilever’s scientists to collect stronger lab metrics so they can develop body wash products with the desired sensory profiles.
Team: Richard (Rui) Wen, Ling Zhang, Sanketh Nagarajan, Jieyu Yao and Jager Hartman
Industry Affiliate: Unilever
Identifying Future Hit Games
Gaming on the Windows platform is a major source of user demand for Microsoft. This team worked to identify future hit games that would evolve quickly from an early audience to a large user base.
To solve this challenge, the team focused its efforts on usage data for gamers who play ‘Steam,’ a popular multiplayer video-game platform. They provided a framework to analyze early trends in game usage to provide insight and make predictions regarding the game’s future popularity.
They used external Steamspy community data, and collected data from a public-facing Application Programming Interface that included statistical information on users who play games on the platform. In the end, they were able to use this data to provide Microsoft with prototypes for several distinct approaches for identifying games that might become hits in the future.
Team: Shashank Shashikant Rao, Cyrus Dinyar Lala, Arman Uygur, Mason Sun and Xin Liu
Industry affiliate: Microsoft
Identifying the Optimal Location for a New Business
Suppose a prospective entrepreneur wanted to open a Chinese restaurant in an area of New York City that has low rents, minimal competition and the potential for high profit? How could that entrepreneur, given these specifications, find an optimal location?
For their project, this team built a web-based application that leveraged machine learning techniques and publicly-available data to identify and recommend an ideal location.
Using a learning algorithm, they divided New York City into 100 neighborhoods. They incorporated the merchant’s objectives and preferences into their model and generated a final recommendation for best location.
Specifically, the team identified five sample factors that a business owner might consider when opening up a business, such as expected profits and costs, demographics, and competition. They scraped publicly-available datasets from Yelp, Foursquare, and NYC Open Data to rate each neighborhood in the city based on these factors and return a recommendation that would satisfy the user’s goals.
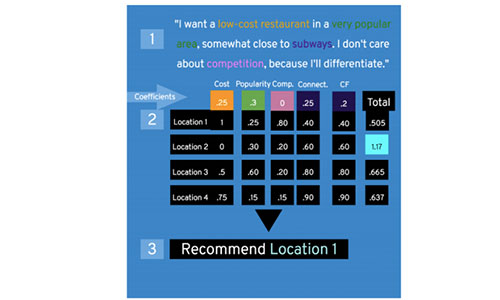
Their algorithm recommended that the optimal location for opening up such a Chinese restaurant would be near to Manhattan’s Rockefeller Center. But more importantly, the algorithm considered a business owner’s different needs and made different predictions depending on those needs. The model can also be used to help merchants find the best location for other kinds of businesses such as laundromats, grocery stories and coffee shops.
“We designed the algorithm in such a way that a business user can re-run it for any business category,” said team member Daniel First. “So if you want to open a laundromat or a bowling alley, you can re-run the app with one line of code. This was a major step forward relative to the current state of the literature.”
Their algorithm was also the first to be designed from the ground up with the business objectives in mind, added First. “Some merchants may want to open a restaurant with profit in mind, while others may want to open a restaurant with their eyes on market share or visibility.
“It was crucial to the success of this project that our algorithms generated recommendations based on a user’s particular objectives,” First said. “And we accomplished that goal.”
Team: Daniel First, Richie Castellanes, Amla Srivastava and Wei Dai
Industry Affiliate: Synergic Partners
Derivative Yield Curve Prediction
Interest rate (IR) data forms the foundational layer of quantitative financial analysis. Market players rely on accurate curves (a curve is made up of a bunch of points and each point can be thought of as a financial time-series) derived from this data to price deals, quantify risk, measure solvency, hedge and more. In an IR curve, some of the points may have data while other points may be missing data. This team set out to predict missing points by using historical data and partially available curve information.
They experimented with multiple models such as univariate models, linear regression and Random forest models. They also applied deep learning with long short-term memory (LSTM) to compare performance of above models in the context of this time series completion problem.
For the purpose of the study, they used the historical 1-month to 30-year YTM from Jan’01 to Sep’17 and compared performances under various settings.
Their resulted show that its best to view this problem as predicting short-term yield and long-term yield separately. While LSTM performs best for short term maturities, they found that a simple ridge regression gives the best results for long- term maturity. They also performed a robustness check, which found that the models help up nicely, with no significant impact on predictive accuracy.
In future, the team said it would like to extend these models to other yield curves and design an ensemble that can be used across asset classes.
One lesson they learned by doing this project was that their industry affiliate preferred their using simple models like Linear regression than with more complicated models. That’s because clients have an easier time understanding the simpler methods.
“So our key takeaway was that simple models are more understandable and therefore provide better results,” said team member Lakshya Garg.“We definitely learned many things over the course of the project,” added Gard, “and will continue to explore and experiment with data-driven methodologies to improve our data offerings.”