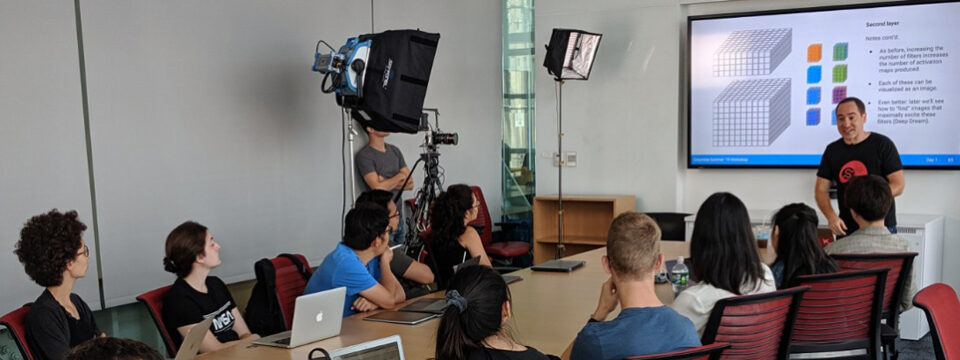
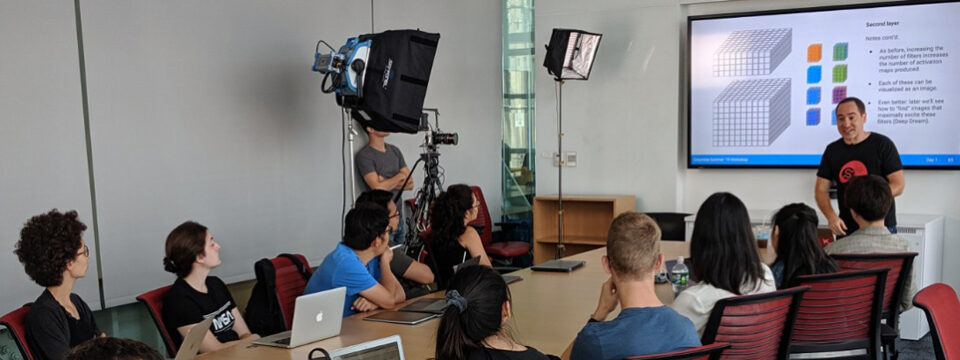
The Data Science Institute has two programs that offer students the chance to work on data-intensive research with Columbia researchers. And both students and the researchers say the programs are extremely valuable.
The first of the two, The Data Science Institute Scholars Program, connects undergraduate and masters’ students with Columbia research professors. The students work closely with the professors, receiving direction and advice that helps them develop data-intensive research skills. Now in its second year, the program introduces students to pioneering academic research whose aim is to solve vexing societal problems. Through enrichment activities, the Scholars Program also aims to foster a collaborative, data-science learning community at Columbia. It began as a summer session, but this year the program, given its success, will extend through the entire academic year.
Also new this year is a branch of the program called Data for Good Scholars, which is only for undergraduate students. Instead of being paired with Columbia professors, these students are paired with officials at nonprofits, community organizations, and government agencies, most of whom are not data scientists nor are they trained in data analysis. The students must take the lead in using data techniques to help solve the various social problems confronting the nonprofits.
Since the launch of the Data for Good Scholars Program in March of 2019, 13 Columbia and Barnard undergraduate students have worked on projects with nonprofit organizations led by Obama Foundation Scholars and New York City agencies.
Both programs were launched by Tian Zheng, associate director for education at the Data Science Institute, who wanted to offer Columbia students more opportunities to work on data-intensive research.
“[The DSI Scholars program and Data for Good Scholars program] resonate well with DSI’s mission to use data for good,” says Zheng, professor and statistics chair at Columbia. “These two programs fulfill DSI’s educational mission while deepening connections between talented students, our renowned faculty and leading nonprofits, who are all working on exciting data-driven research.”
Vince Dorie, an associate research scientist at the Data Science Institute who co-directs the programs with Zheng, says an inspiring aspect of the Data for Good program is how much the students are learning about real-world problems, and how much the nonprofit agencies and officials are learning about good data science practices from the students. “It’s really proven to be an invaluable experience,” says Dorie, “for everyone involved.”
DSI member Galen McKinley is a professor of Earth and Environmental Sciences at the Lamont Doherty Earth Observatory. She studies the role that the ocean plays in the relationship between emissions and climate change by serving as a carbon sink – or by absorbing large amounts of excess carbon dioxide. Her research is data intensive, and she’s had DSI scholars assist her in the lab.
“The DSI scholars program is very good because it has inspired collaboration between my group and data science students looking for problems to solve,” says McKinley. “I have learned about how modern data science approaches can benefit my research, and the students have learned about jumping into a new research area that they know little about. They are learning about consulting for a new client, and I am getting the benefit of their expertise – just as in a client-consulting arrangement in the real world.”
Cory Abate-Shen directs a lab that seeks to understand how normal mechanisms of cellular differentiation are co opted in cancer. She also had a DSI scholar assist in her lab.
“The program connects students who want experience with laboratories like mine so that they can experience a lab setting and understand the real-world applications of the work that they are being trained in,” she says. “We had a wonderful student who really became a part of our laboratory and is still working with us and contributing to an important project.”
Here are brief descriptions of the projects the DSI scholars have worked on:
Measuring Liberal Arts: Creating an Index for Higher Education with Peter Bearman
Through anecdotal evidence of departments shrinking and fierce competition for professorships, there is a general sense that the role of the liberal arts in higher education is undergoing a dramatic shift. In order to make this sense or suspicion more precise, researchers first must establish a definition of what constitutes a liberal arts education as well as methods of measuring how widely it is taught. To that end, this project is developing a novel collection of text-based school data taken from syllabi and course catalogs. The data will be used to build a multi-dimensional measure of the status of liberal arts education in America.
Student: Samuel Deng
Learning Representations and Patterns in Mathematical Proofs with Nakul Verma
Until they become accustomed to the necessary way of deductive reasoning, students often find it difficult to argue in the manner of a mathematical proof – where conclusion follows from the premises by a chain of logical statements. By analyzing student homework assignments and their solutions to problems, however, researchers can discover patterns of mistakes in their reasoning. And those patterns can then be used to adjust instruction and help develop this important but often elusive mathematical skill.
Students: Yanda Chen, Michel Vazirani
Blockchain Anomaly Detection with Siddhartha Dalal
Blockchain is poised to upend a number of industries through the promise of decentralized digital ledgers. The transparency associated with this technology, however, can only build trust if participants know what patterns of historical transactions are trustworthy. This project has collected a large dataset from a cryptocurrency blockchain and is developing methods for detecting anomalies in transactions based on social networks, graphs, and machine learning methods.
Students: Siddhanth Sabharwal, Xiaoqi Wang
Visualization of Continuous Health Data Measurements with Sam Sia
The shortcomings of continuous internal sensing devices – more invasive and expensive than external monitors – has limited the impact of predictive analytics in healthcare. To overcome this deficiency, this research team has developed a wearable device capable of continuously measuring blood levels of glucose and electrolytes without requiring implantation. This technology can augment the functions of currently existing activity sensors to better monitor chronic conditions such as diabetes and arrhythmias, as well as facilitate preventative medicine. This project seeks to take data from sensors, integrate the data with existing wearables and evaluate their combined discriminatory power.
Student: Christian Pascual
Data Science for Better Health with Max Topaz
An aging population and the adoption of electronic health record systems has resulted in an explosion of data about elderly home health care, including structured content, free text clinical notes, and recorded patient-provider phone conversations. The goal of this project is to use this data to build predictive models that help identify patients who are at risk of poor health outcomes such as hospital admission or falls.
Students: Adrian Blanco, Le Chang, Liheng Fu, Jie Li, Huy Nguyen, Mingming Song, Alyssa Vanderbeek, Jincheng Xu, Xinyu Zang, Xiaofan Zhang, Chuhan Zhou
Looking for the Weird in TESS with David Kipping
Using a novel algorithm to detect “weird” signals in photometric time series, such as those taken by NASA’s Kepler Mission, this project aims to detect anomalous signatures in NASA’s new Transiting Exoplanet Survey Satellite, known as TESS. Possible weird signals include analogs to Tabby’s Star, interacting binaries and perhaps even techno-signatures.
Student: Joheen Chakraborty
Enhancing Self-Directed Learning Opportunities with Gary Natriello
Self-directed learning remains an important component in the democratization of knowledge, and has only been enhanced by advances in information technologies. In this project, a team is analyzing data from library applications/systems developed by the Education Laboratories at Columbia Teachers College that are designed to facilitate autodidacticism, such as the PocketKnowledge system for online archival or Vialogues for video discussion. The team is also creating visualizations that highlight the most efficacious tools.
Students: Ameya Karnad, Henry Williams
Random Forest vs. Neural Networks for Estimating the Ocean Carbon Sink with Galen McKinley
The ocean plays an essential role in the relationship between emissions and climate change by serving as a carbon sink –absorbing large amounts of excess carbon dioxide. Direct measurements of surface pCO2 are infeasible due to the enormous costs associated with surveying the entire ocean. Satellite data, however, can be used as a more cost-effective proxy. This project uses machine learning techniques to extrapolate from sparse but fully specified observations to full coverage fields using auxiliary data that can be measured remotely.
Student: Monica Yan
Distance Metric Learning in Hyperbolic Spaces with Nakul Verma
Encoding symbolic data such as words and networks in Euclidean spaces is inefficient but commonly done to fit those kinds of data into the traditional tools of data science. For example, the vector space representation of a sentence consists of counts of the distinct words in the sentence but also a large number of zeros, one for each word that is in the dictionary but not otherwise present. By operating directly on social network and lexical data, the project will use machine learning to develop a more effective notion of distance between entities in the original symbolic space – also known as a hyperbolic space. By avoiding the unnecessary dimensional expansion induced by the Euclidean representation, measures on the hyperbolic space should be able to help in classification tasks by reducing computation and increasing performance.
Student: Max Aalto
Advancing Public Health Monitoring and Analytics in New York City through Development of a Master Person Index with Jeanette Stingone
The New York City Department of Health and Mental Hygiene aggregates data from a large number of sources with the aim of protecting and promoting the health of all New Yorkers. Linking these data sources has proven to be prohibitively intensive, driving the development of a master person index (MPI). This project enhances the MPI tools and services, as well as evaluates different machine learning matching models.
Student: Jinhao Zhang
ArXivLab: A Platform for Developing and Evaluating Exploratory Tools for the Scientific Literature with Kriste Krstovski
Through ArXivLab, this project aims to develop next-generation recommender systems for scientific literature using statistical machine learning approaches. In collaboration with ArXiv, the team is developing a new scholarly literature browser that will be able to extract knowledge implicit in the mathematical and scientific literature, offer advanced mathematical search capabilities, and provide personalized recommendations.
Student: Jin Woo Won
Towards An Understanding of the Visual System’s Architecture in the Human Brain
The visual cortex, the part of the brain responsible for vision, has a very distinct organization. It is unclear, however, as to which factors shape this distinctive architecture. Feasible factors could include internal phenomena such as energy constraints or external phenomena such as the actual composition of the visual world. This project employs computational modeling and artificial neural networks, as well as a famous algorithm from the artificial intelligence field to understand how and why the visual cortex’s architecture emerged with such a distinctive organization.
Student: Lucas Stoffl
Measuring Broadband with Henning Schulzrinne
The Federal Communications Commission (FCC) and the U.S. Census routinely publish data on national Internet availability across the United States. The data is detailed and granular, covering census blocks and counties and states. The goal of this project is to answer questions based on the available data such as How reliable is Internet access? Who is deploying fiber where? ‘Can we predict reliability of different technologies? and Can we predict the deployment of fiber?
Student: Tanya Hao
Training a Deep Neural Network on Large-scale Brain Imaging and Cognition Data with Jiook Cha
The goal of this project is to develop and validate a model for predicting a child’s emotion, cognition, and social development. The team will use 3D convolutional neural networks trained on brain imaging data to develop the model. The goal is to design a scalable deep neural network that will help find the underpinnings of the brain’s cognition and emotion.
Student: Seungwook Han
Discover Novel Regulators of Macrophage Efferocytosis by Genome-wide CRISPR Screening with Hanrui Zhang
Defective efferocytosis, the phagocytic clearance of apoptotic cells by macrophages, is the cause of many human diseases including tumors, autoimmune diseases and atherosclerosis. Many key regulators of efferocytosis have been identified but a systematic approach to map these regulators in an unbiased manner on a genome-wide scale has not been developed. This project uses genome-wide CRISPR screening to discover regulators of macrophage efferocytosis. The team will draw upon public data sources and use data visualization techniques to illustrate the data.
Students: Jianyou Liu, Jiayi Shen
Analysis and Prediction of Opioid Outbreak Clusters with Jeffrey Shaman
This team will investigate how deaths and hospitalizations from opioid overdoses cluster across space and time in the U.S. Once the drivers of opioid clustering are established, the team will develop forecasting models that will assist in public-health responses, such as distributing Naloxone to the region’s most in need of it.
Students: Mary Fangyuan Liu
Discovery of Genes Associated with Progression of Bladder Cancer with Cory Abate-Shen
We have been studying bladder cancer in a mouse model of the disease and we are seeking to understand the molecular features of the mouse models as they relate to human bladder cancer.
Student: Rachel Tsong
Injury-Disease Interactions in Older Adults with Guohua Li
The interaction between injury and disease is complex, mutually causative, and difficult to model. For instance, patients with Alzheimer’s disease or Parkinson’s disease are known to be at heightened risk of hip fractures from falls; and in turn falls among these patients can drastically worsen the trajectory of the disease. This project thus aims at uncovering the Gestalt of the relations between different injuries and different diseases by analyzing a large data set on older people compiled by the National Center for Health Statistics and the Centers for Disease Control.
Students: Adina Zhang
Outcomes of Multi-visceral Resections in Colorectal Cancer with Sung Kwon
Fundamental to oncological surgery – and often effective at eliminating certain kinds are cancer – are balancing the risks of postoperative complications with the survival benefits that accrue to patients who have surgery. This project will evaluate the safety and long-term survival data of patients with T4 colorectal cancers following multivisceral resections, and compare that to data on patients who received chemotherapy or radiation.The team will use a multi-institutional national cancer database.
Students: Dayoung Rebekah Yu
Social Media Recommendation Engine Bias with Sid Dalal
More than 70 percent of content consumed by YouTube is driven by its recommendation engine. Some observers have claimed that the engine tends to recommend sensational videos, which can influence or radicalize viewers. By radicalization, the team means recommended videos from both the far right and the far left as well as those espousing radical theories that contradict widely accepted facts. The goal of this project is to test these claims scientifically by following a sequence of recommendations and using natural language processing to divide the videos into classes.
Students: Jaidev Shah
Factor Modeling for Portfolio Management by Minsu Yeom, supervised by Kriste Krstovski
Factor investing is a widely accepted way of gaining a systematic exposure to equities. Inspired by topic modeling in natural language processing, this project aims to group stock returns showing co-movements and generate their distributions through nonnegative matrix factorization, essentially generating a distribution on distributions of factor returns. By selecting stocks that are expected to perform well according to this distribution, it should be possible to construct a factor portfolio that meaningfully outperforms the market.
Student: Minsu Yeom
Recent Data For Good Projects
Developing Eye-tracking Methods for Cost-Effective Reading Assessments in Paraguay with Obama Scholar Gabriela Galilea, who runs the company, Okimo
Current best-practices for assessing a student’s reading ability involves a time consuming and costly session with a reading education specialist. Reading levels throughout Paraguay remain uncommonly low for Latin America and individualized attention – particularly in rural areas – is infeasible. Our goal is to develop a low-cost alternative using eye-tracking technology, audio recordings, and machine learning that can prescribe an educational intervention using a vastly simplified assessment.
Students: Noah Chasek-Macfoy, Helen Jin, Daniel Knop, Lihao Xiao, Jennifer Wu
Obtaining Accurate Population Estimates of Indigenous Peoples in Rural Colombia with Ana Maria Gonzalez Forero, an Obama Scholar,and her group, Fundación para la Educación Multicultural
By law, legal protections including rights to land have been extended to indigenous people in Colombia. Many of these populations, however, exist in rural areas that are hard to assess. Official government estimates aren’t incentivized to be accurate and contain disparities across ethnic minority groups. As a means of remedying this, this team will create population estimates derived using measures of urbanization – data from field work that assess where people live based on roads and buildings. The team will use maps, visualizations, and other estimates derived from urbanization data. That data will be compared to official government accounts and petitions filed by residents for legal protection.
Students: Vivek Kantamani, Rea Rustagi, Xinyue You, Amy Zhang
Predicting Cost Overruns and Delays in NYC Capital Projects with Terri Mathews, Senior Policy Adviser at the NYC Department of Design and Construction
The large scale, cost, and complexity of capital improvement projects result in a great number of potential causes that can lead to a project missing a deadline or coming in over budget. Using data from the NYC Open Data portal, this project aims to first roughly categorize what kinds of projects are subject to routine overruns, which in turn can be used as impetus for more detailed data collection and analysis. Ultimately, the team’s goal is to determine when projects are in danger of missing deadlines and be able to address problems before they occur.
Students: Erinn Lee, Jady Tian, Lara Yener