Nudging New York: Using Data Science to Increase Healthcare Access in Underserved Communities
February 16, 2019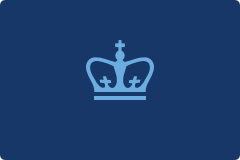
Project: Nudging New York: Using Data Science to Increase Healthcare Access in Underserved Communities
Team members: Kai Ruggeri, Department of Health Policy and Management, Mailman School of Public Health; Marianthi-Anna Kioumourtzoglou, Environmental Health Sciences, Mailman School; and John Paisley, Department of Electrical Engineering, Columbia Engineering
Federally funded healthcare centers provide medical care to more than 20 million Americans who live in impoverished areas. Yet in many of those areas, nearly half the patients who make medical appointments at the centers are unable to keep their appointments. Data show that patient no-shows, and the missed opportunities for needed medical care, place an enormous health burden on disadvantaged communities. No-shows, for example, increase the likelihood that patients will eventually visit emergency rooms and be hospitalized for conditions that could have been caught and treated at clinics. As such, even small decreases in no-show rates at community healthcare clinics will improve the health of vulnerable populations and reduce the nation’s medical costs.
This research team intends to do precisely that – reduce missed appointments at community clinics – by using big data and Bayesian machine learning techniques to understand why patients miss appointments and what can be done to help them keep them. The researchers have partnered with the Community Healthcare Network, a federally funded clinic in New York City serving disadvantaged communities.
To mitigate this problem, the team will evaluate behavioral data relating to no-shows from clinics in the Community Healthcare Network, spanning Brooklyn, the Bronx, Manhattan, and Queens. They will also explore common environmental barriers to care, such as transportation, childcare, translation services, and appointment times. Once the team has evaluated patients’ behavioral and environmental data, as well as data from emergency departments at partner hospitals, it will use a technique known as “nudges” to help them keep their appointments. Nudges are behavioral interventions that encourage optimal choices without restricting options. They can be applied in a variety of ways, such as providing more salient information to patients, or presenting more clearly the importance of attending regular checkups in the long-term. What is most important is that the nudges are personalized, as the most effective interventions are those that address the specific needs of individuals.
There is room to improve on previous attempts by clinics to reduce no-shows, which may further benefit from machine learning approaches using big data. This will be especially effective through understanding patient needs and creating the proper interventions to help patients keep appointments, says Kai Ruggeri, Assistant Professor in Health Policy at the Mailman School of Public Health. And nudges have shown particular promise in helping patients.
“By integrating and evaluating multiple sources of clinical, behavioral, and environmental data, and then matching the most effective interventions to the right groups of patients, our team hopes to reduce no-shows and avoidable visits to emergency departments,” adds Ruggeri. “By implementing Bayesian machine-learning methods to better understand patterns of behavior in these groups, we should be better able to design nudges that increase healthcare access for the most vulnerable New Yorkers. And if we do it right, the system we create can be used at community clinics all across the U.S. to improve healthcare while reducing cost.”
— Robert Florida