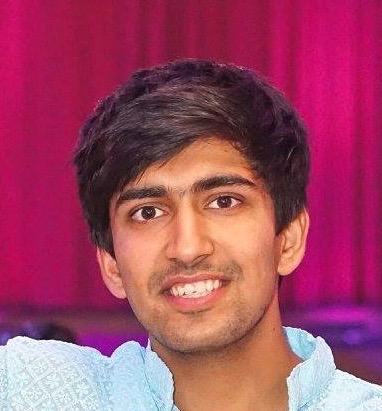
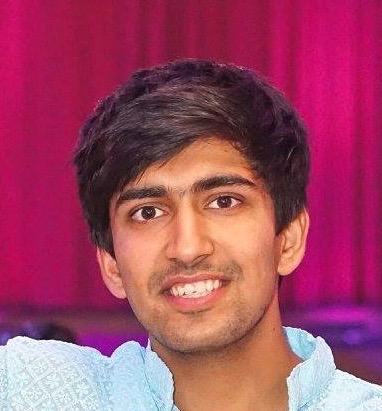
Pranjal Bajaj, who graduated from DSI’s master’s program in May, will begin working this summer as a data scientist at Boston Consulting Group GAMMA. There, he will use the data science skills he learned at DSI to solve an array of business challenges for the group’s clients.
“It’s a dream job,” says Bajaj, “in that I’ll have opportunities to use techniques such as machine learning and deep learning to help BCG’s clients implement data-driven solutions to business problems. I’ll also get to see how private corporations operate, which will be interesting since until now all my work has been in the nonprofit world.”
Bajaj’s work for nonprofits has been significant and helped him get his job at BCG. While he was in the master’s program, for example, he participated in UChicago’s Data Science for Social Good Fellowship , where he was part of a team that worked directly with the Portuguese government to design a machine learning platform. It enabled the Institute of Unemployment and Professional Training of Portugal to predict a citizen’s risk of becoming unemployed for longer than 12 months, categorized as long-term unemployment.
The institute is now using the platform to create preventative interventions to help those at risk of long-term unemployment to develop their skills and find work. The tool will potentially affect a third of the Portuguese population, the percentage of the population for which the team had data. The team’s work was accepted at the Neural Information Processing System’s (NIPS) AI for Social Good Workshop as a short paper and a poster presentation.
In this Q&A, Bajaj discusses the fellowship, his new job and his passion for using data science to solve problems and improve society. He also talks about how he transitioned from having studied economics and law at Cambridge University in England to studying data science at Columbia.
How did you hear about the Data Science for Social Good Fellowship?
A friend found the opportunity online and shared it with me, telling me that I would be perfect for this. I was hesitant to apply at first since the fellowship mainly admitted Ph.D. students in technical fields, while I had only started programming just a few months prior to applying in January 2018. However, my background in the social sciences and subsequent exposure to machine learning at DSI helped me succeed in the interviews and also prepared me well for the fellowship.
What is the aim of the fellowship?
The Data Science for Social Good Fellowship run by the University of Chicago is an initiative that connects data scientists to governments, NGOs and other such organizations to address high-impact issues facing societies. The fellowship is run in multiple locations each year and locations change each year, depending on where the organizations that UChicago decides to work with are based. I was in Lisbon, Portugal, for the whole duration: three months.
You don’t speak Portuguese, so how did you communicate with officials from the Portuguese government?
We had weekly meetings with the government, 90 percent of which were in Portuguese. One of my teammates was Brazilian so Portuguese was her native language; the other two spoke Spanish and hence understood a little Portuguese. The three of them would transcribe English translations for me in real time on Google Documents during meetings to keep me in the loop. It was incredible teamwork and I did pick up some Portuguese this way!
Is unemployment a major problem in Portugal? And is data science a good tool with which to contend with the problem?
Unemployment is one of the most pressing economic issues in post-crisis Portugal. The economy has been through two major recessions in the past 10 years, with unemployment peaking to around 16-17 percent during the height of the crisis in 2013. An even more severe problem is that of long-term unemployment: 50 percent of the unemployed stay unemployed for longer than 12 months; this is the 5th highest rate in Europe. The Institute of Employment of Professional Training of Portugal is responsible for re-skilling individuals who are unemployed and helping them find work.
Data science is especially useful in this area since predictive models allow us to identify individuals at the highest risk of becoming long-term unemployed and channel the employment institute’s limited resources to those most in need. In fact, several European governments, including Portugal, already use risk assessment tools for this purpose.
What was the data science solution your team produced? Do you think you succeeded?
The government had a model in place that gauges long-term unemployment, and our role was to build a more accurate model, which is also more useful for the end-user, i.e., case workers who work with unemployed individuals to prepare them for jobs. By the end, we were able to build a model that provided a significant lift compared to the model the government had in place while keeping our results interpretable. We used an XGBoost model that allowed us to make more accurate predictions, and used SHAP values to understand how an individual’s attributes affect their respective risk profiles. For example, an individual with four years of work experience has a 12 percent lower risk score than someone who has no work experience, given that all the other attributes for both these individuals are the same.
Our biggest accomplishment was getting our work accepted into NIPS in their AI for Social Good Workshop last December. Our paper discussed how we were able to significantly bridge the accuracy vs. interpretability trade-off in our project. Another plus is that our work from the fellowship is currently being implemented by the government. So, in a way, I do believe that we were successful.
Did your team present at the NIPS conference?
We completed the Fellowship project in August. Then Rayid Ghani (founder of the fellowship) suggested that we apply to the NIPS AI for Social Good workshop. Led by our technical mentor, we put together a paper summarizing our work and submitted to NIPS in October; we got an acceptance in November. Our technical mentor for the project, who has been working with the government since August to implement our project, presented the poster at NIPS.
Was the fellowship a good experience? Did you learn from your peers?
I felt really lucky being around some of the smartest and most motivated group of people I have ever met. A majority of the fellows came from top Ph.D. programs across the world, covering almost every continent. There was not just geographical diversity in the group but also great cultural and academic diversity, which provided an excellent breeding ground for critical dialogue and learning via discussion.
One thing that really brought us close was our resolve for doing good and doing it the right way. Most fellows had a strong sense of empathy, which explains why they were part of a data for good fellowship. And despite being technologists, most fellows saw technology as a tool that, if used well, can help create social good. Being a part of a group of such caring and devoted people was everything I was looking for in a job.
And from a technical perspective, I learnt everything I could ask for and more. I went from the beginning phase of a machine learning pipeline, where we spent a four weeks just making sense of the data, to building a dashboard that can generate interpretable predictions at the click of a button by the twelfth week. Overall, what’s better than spending 8 hours of the day doing something you love and the rest of your time exploring one of the most beautiful countries I’ve ever visited.
You studied economics and law at Cambridge University in England. Did that background help you understand the problem of unemployment? And does your background inform your understanding and use of data science?
Yes definitely. I was quite familiar with the domain we were applying machine learning to and it helped me get my team up to speed with a lot of concepts and the lingo. My training in economics came in especially handy during the feature engineering stage of the project as we decided to include macroeconomic features in our dataset such as fixed effects (recession or not), consumer confidence, inflation, interest rates, etc., and we built these features at different denominations of the geography (such as district, city state, country level).
Most of my work before coming to Columbia was in the social sciences, and learning data science helped me see the transformative potential it holds to improve social science research and public policy. I see data science as a means to an end, the end being to improve society by combining it with other domains such as social sciences, which happens to be my area of interest.
You also worked on a data-related social good project at Columbia Law School. Can you discuss that?
I assisted Columbia Law School Professor Colleen Chien on a project that studies biases in pre-trial flight risk assessment systems (risk of defendants failing to appear for trial), which judges use to decide which defendants should be incarcerated before trial and which of them should be granted bail. It’s a topical and high impact project that can potentially affect the liberty of thousands of defendants. I will continue to work on this project with Professor Chien as a DSI alum.
You’re an avid reader, so what books have inspired your interest in ethics and data science?
Automating Inequality by Virginia Eubanks is a book that my technical mentor from Data Science for Social Good advised me to read. Coincidentally, as I started working for Prof. Colleen Chien a few weeks later, she handed me her copy of the book, telling me that this was prerequisite reading for our project. The book really changed how I see the impact of machine learning on people. It made me realize that the effect of machine learning is very much tangible and the smallest of biases, many of which come from the data, can spiral out of control and have unprecedented consequences for people if not dealt with intelligently. The book singles out case studies to show just how complex a role predictive analytics plays in the public policy space while evoking a sense of urgent action from her readers. Another equally amazing book to look at in this area is Weapons of Math Destruction by Cathy O’Neil, which will be my next read!
What were the highlights of DSI for you?
In terms of coursework, taking Machine Learning with Professor John Paisley and Applied Machine Learning with Professor Andreas Mueller were great experiences. A combination of these courses really sets you up for industry as you not only develop the theoretical underpinnings of machine learning but also learn how to apply it from the perspective of a practitioner. Other extremely relevant and interesting classes I took were Bayesian Statistics with Prof. Andrew Gelman and Algorithms for Data Science with Prof. Eleni Drinea.
In my final semester I took the Capstone Project, which allowed me to apply the skills I had developed at DSI and get a feel for what it’s like to work in industry. I also spent my final semester working as a Course Assistant for Prof. Mueller, for his Applied Machine Learning course, which was an experience in itself given his unfathomable contributions to the open-source machine learning community. Holding office hours for students and sharing my knowledge and intuition of applying machine learning to real world projects that I had developed over the past year through this class and my work at the fellowship were great!
Are you excited to start your new job this summer?
After the Data Science for Social Good fellowship, I was determined to work in data science consulting since I realized that to succeed in a career in data science I need to strengthen my intuition of applying machine learning to different business domains. BCG Gamma is one of the best places to get this exposure while creating measurable impacts and, yes, I’m really excited to start work there!