Biostatistician, DSI Member Jeff Goldsmith on the Importance of Data Science and Public Health Collaborations
April 13, 2020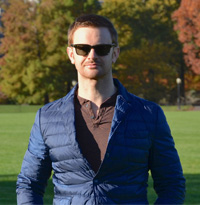
“I use my quantitative training in ways I hope will have an impact on society, and data science has emerged as a critical component of biostatistics and public health research.” – Jeff Goldsmith
The coronavirus/COVID-19 hadn’t reached pandemic status during Columbia University’s Data Science and Public Health Summit in mid-January. Now, it is the public health challenge of the 21st century.
“This pandemic has shown the necessity of using data-driven approaches to contend with a public health challenge of an enormous magnitude,” says Jeff Goldsmith, an associate professor of biostatistics at the Mailman School of Public Health and member of the Data Science Institute. “I’m proud of my colleagues at Mailman and at DSI, many of whom are working tirelessly to provide the insight and expertise needed to save lives across the world.”
Goldsmith majored in mathematics as an undergraduate at Dickinson College and discovered biostatistics while considering fields to study in graduate school. It was the perfect field for him as biostatisticians, he learned, use quantitative methods to solve problems and benefit society. He completed the doctoral program in biostatistics at Johns Hopkins University, where his dissertation focused on statistical methods to understand high-dimensional structured data.
What originally drew Goldsmith to DSI when he arrived at Columbia in 2012 was the insistence on using “data for good.” Today, he is part of an interdisciplinary team awarded a DSI seed fund grant to identify the main sources of air pollution in India. He also teaches a course developed through The Collaboratory at Columbia University called Data Science in Biostatistics, which entered Mailman’s curriculum in 2016. The course has grown in popularity and its enrolled students major in biostatistics, epidemiology, or environmental health.
Goldsmith is most vocal about his interest in developing ethical guardrails to guide data science research and teaching as machine learning and AI algorithms have introduced biases that accentuate ethical dilemmas. He discusses how gender bias crept into Google Translate, which was initially heralded for its efficiency. It soon became apparent that the translator was gender biased, with translations including “he is a soldier; she is a teacher; he is a doctor; she is a nurse.”
“When you train an algorithm in a way that seems to give good answers, but you really don’t understand it, you can reproduce biases, which is what happened here,” Goldsmith says.
Data scientists do not usually conduct research on human subjects. As a result, he says, data science lacks a governing body to establish ethical guidelines for the field. He suspects some of the ethical principles he abides by as a public health researcher can be applied to data science.
“For us in public health, whether you are doing data science research or clinical trials you are focused on ethics,” Goldsmith says. “As data science moves into more and more fields now, it’s facing increasing ethical challenges. I’m studying to see if I can apply some of the guidelines we use in public health to develop foundational ethical principles for data science.”
— Robert Florida