Data Science Institute Seed Grants Support Interdisciplinary Research Across Columbia
January 8, 2020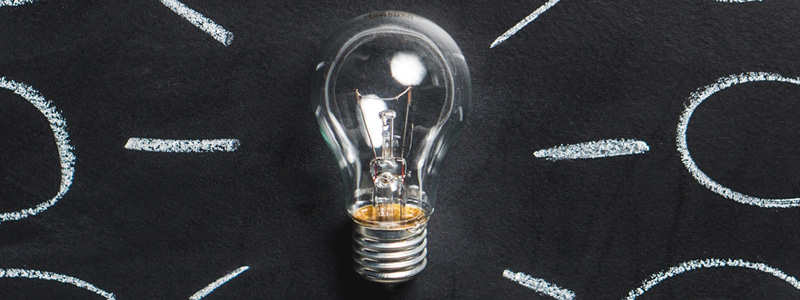
The Data Science Institute (DSI) at Columbia University has awarded 2020 seed grants to research teams whose projects merge data science with traditional fields to solve pressing societal problems. DSI’s Seed Funds Program supports new collaborations to forge long-term relationships among faculty in different disciplines and use data science to transform all fields across Columbia. Two of this year’s six seed grants are jointly sponsored by Columbia’s Irving Institute for Cancer Dynamics.
The following research teams and projects have received 2020 awards.
Artificial Intelligence-Assisted Identification of Child Abuse and Neglect in Hospital Settings with Implications for Bias Reduction and Future Interventions
Max Topaz (Nursing), Aviv Landau (DSI), Desmond Patton (Social Work)
Child abuse and neglect is a social problem that has reached epidemic proportions. The broad adoption of electronic health records in clinical settings offers a new avenue for addressing this epidemic. This team will develop an innovative artificial intelligence system to detect and assess risk for child abuse and neglect within hospital settings that would prioritize the prevention and reduction of bias against Black and Latinx communities.
Gender and Racial/Ethnic Inequality in High-Skilled Labor Market: Gaining New Insights from Online Resume and Reviews Database
Yao Lu (Sociology), Kriste Krstovski (DSI)
This research team will combine new sources of labor market data, which include online resumes and employee reviews, with data science methods to identify factors and environments that shape gender and racial inequality in high-skilled labor market. The team will chart long-term career trajectories of a large number of high-skilled American workers and examine gender and racial variations; and construct measures of company environment, especially that pertains to gender and racial equity, and assess its consequences for the career path of different groups of skilled workers.
Detecting and Attributing Spatiotemporal Variations in Sources of Ground-level Air Pollution with a Modeling Testbed for Integrating Multiple Noisy Satellite Datasets
Arlene Fiore (Earth and Environmental Sciences, Lamont-Doherty), Daniel Westervelt (Lamont-Doherty, NASA), Jeff Goldsmith (Public Health/Biostatistics), Marianthi-Anna Kioumourtzoglou (Public Health/Environmental Health Sciences), Ruth DeFries (Ecology, Evolution and Environmental Biology), John Wright (Electrical Engineering)
This project seeks to develop methods to extract patterns from multiple datasets and thereby identify the dominant sources of air pollution across India and how they vary in space and time. The proposed work is a step toward the overarching goal of informing effective clean air solutions and reducing public health burdens associated with exposure to air pollution in India.
Interpretable Microbiome Dynamics in Liver Transplant Recipients
Itsik Pe’er (Computer Science), Anne-Catrin Uhlemann (Irving Medical Center/Infectious Diseases)
This project will develop methods for temporal analysis of gut microbiome compositions to better define the risk of infections in liver transplant recipients. The project team will integrate existing coarse resolution data with newly collected deep metagenomics and metabolomics data.
Modeling the Dynamics of Young Onset Colorectal Cancer Using Big Population Data
Wan Yang (Epidemiology), Mary Beth Terry (Epidemiology), Jianhua Hu (Biostatistics), Piero Dalerba (Pathology and Cell Biology)
Using multiple nationally representative large-scale exposure and cancer incidence datasets, this project will build a novel model-inference system to study the dynamics of colorectal cancer, test a range of risk mechanisms over the life course, and identify key risk factors underlying the recent increase in young onset colorectal cancer incidence in the United States to support more effective early prevention.
Probabilistic Modeling of Intercellular Interactions that Drive Ferroptosis Susceptibility of Therapy-resistant Cancer Cells
Elham Azizi (Biomedical Engineering), Jellert Gaublomme (Biological Sciences), Brent Stockwell (Biological Sciences)
This project will leverage machine learning techniques to combine two types of single-cell data modalities with the goal of achieving a more comprehensive characterization of heterogeneous cell states in the tumor microenvironment. Specifically, the team will develop probabilistic models to elucidate the role of intercellular interactions in driving susceptibility of treatment-resistant mesenchymal tumor cells to a newly discovered ferroptotic vulnerability, which could offer a therapeutic avenue to prevent survival of these cancer cells that are prone to metastasis.
Data-Driven Discovery of Latent Structure in Human Information Demand
Jacqueline Gottlieb (Neuroscience), Vince Dorie, Associate Research Scientist (Data Science Institute)
As information – and misinformation – become increasingly overwhelming, it is increasingly important to understand both how humans decide which sources of information to consult and how that choice relates to their decision-making strategies. In this project, online behavioral data will be collected from a large sample of participants, using a battery of tasks that probe different theories of how information is prioritized and used. This combined data set will allow an analysis of the latent factors that shape human-information demand while also unifying those theories. This unification can then be used to develop strategies to increase or decrease the frequency of information solicitations, for example helping people on the internet click their way to factual information, or helping those suffering from anxiety disorders to reduce uncontrollable ruminations.
Three research projects and four education projects, moreover, will be funded through the Columbia-IBM Center of Blockchain and Data Transparency, a joint initiative with the School of Engineering and Applied Sciences.
Economics of Blockchain Adoption
Jay Sethuraman and Garud Iyengar (Industrial Engineering and Operations Research)
Pathways to Enabling and Ensuring Legal and Regulatory Certainty, Transparency and Security for Blockchain and Smart Contract Use in the Emerging Crypto-Economy
Leon Perlman and Robert Farrokhnia (Business)
Coded Blockchain for Internet of Things
Xiaodong Wang and Alexei Ashikhmin (Electrical Engineering)
Blockchains and Applications
Alexandros Biliris and Eran Tromer (Computer Science)
An Introduction to Blockchain Technology
Xiaodong Wang (Electrical Engineering)
Foundations of Blockchain
Tim Roughgarden (Computer Science)
Introduction to Blockchain and Cryptocurrencies
Gur Huberman (Business)