DSI Alumni Download: Q+A with Emily Cassell, Data Engineer at Shutterfly
March 3, 2020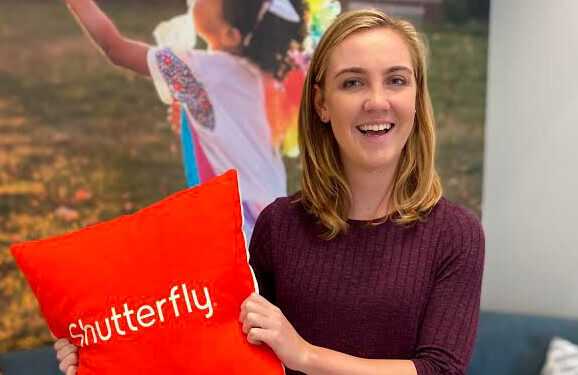
Data engineer Emily Cassell taught herself the programming language R to run regressions on bitcoin price while she studied economics and government at Claremont McKenna College. It was her first experience with data science and she was hooked. Here, the DSI alumna discusses how that hook brought her to Columbia, and how earning a master’s degree paved the way to her current role at Shutterfly, the picture-sharing and photo products company.
How did you land your job at Shutterfly?
Scrapbooking has always been a guilty pleasure of mine, so I immediately recognized Shutterfly’s brand when I saw a data engineering job posting on LinkedIn. From there, I passed two phone interviews before being asked to interview onsite in Redwood City, California. Though I demonstrated my problem solving skills and technical ability during the interviews, I think what set me apart was my interest in Shutterfly’s products and mission in addition to my interest in the data engineering work.
Would you mind describing one of your recent projects for us?
Our marketing data team is undergoing a database migration. All the company’s data are being moved from one provider to another. This is being done to reduce costs as the company grows as well as to improve performance. My projects revolve around working with the analytics team to ensure their dashboards work with the new database structure. As a data engineer, you have to be cognizant of how the data you are moving will be used by the data scientists and analysts. By understanding how the data will be used, I can make sure that the right data is getting to the right people.
How is a data engineer different from a data scientist?
Data engineers ensure that data gets from where it is generated—websites, satellites, self-driving cars, etc.—to where it can be stored and used for analysis. A user may not realize it, but a lot happens between clicking a link on a page and ensuring that that data point enters a data scientist’s machine learning algorithm. Data engineers make sure that a company’s data is stored efficiently and accurately so that it can be used for analytical purposes.
How did you develop a passion for data science?
For my undergraduate thesis, I taught myself R to run regressions on bitcoin price and volume data during Brexit. It was an amazing experience to independently collect and process data to answer questions for my own curiosity. I also wanted a deeper education on the technical aspects of machine learning and data science to supplement the theory I learned as an economics major. Additionally, after a year of working in finance, I was concerned that my desire to complete long trails would force me to choose between my career and my passion for long-distance hiking. When I later understood the culture of tech companies, which focus on one’s work ethic and production rather than face time in the office, I was further motivated to pursue a career in tech. With greater flexibility and work-from-home options, this summer I was able to complete Utah’s 104-mile Highline Trail.
Why did you decide to come to Columbia’s DSI for your master’s degree?
The location of the Data Science Institute at Columbia University, a top school in New York City, originally drew me to the program. I began my journey as a part-time student while working in finance as a market’s analyst for the Federal Reserve, the career opportunity at the Federal Reserve that brought me to NYC in the first place. After a year of balancing work and school, I was excited by the data science material I was learning through DSI. I knew that the fast-paced world of technology was more in line with my long-term career objectives and decided to pursue my degree on a full-time basis.
Describe a highlight of your DSI experience.
The best part for me was the people. There are so many talented people who come to DSI. I learned just as much from my classmates as the curriculum and I’m excited to see what amazing things our class accomplishes.
Do you have any advice for students thinking of studying data science?
Don’t count yourself out if you come from a nontechnical background. I had no formal coding education before starting this program, and if it weren’t for the encouragement of friends and mentors, I may never have started this technical journey. It wasn’t easy to start as a beginner in a new field, but with dedication and relentlessness, I have been able to compete with students who have been studying this for twice as long. Additionally, understand that DSI doesn’t just prepare you to be a data scientist. The skills you can learn here are applicable to many different jobs and industries, both technical and nontechnical. I came to DSI hoping to make a career change, and was impressed to find how many different fields are open to students with strong technical and statistical backgrounds.
— Robert Florida