Data Science Institute Seed Grants Support Interdisciplinary Research Collaborations
January 19, 2021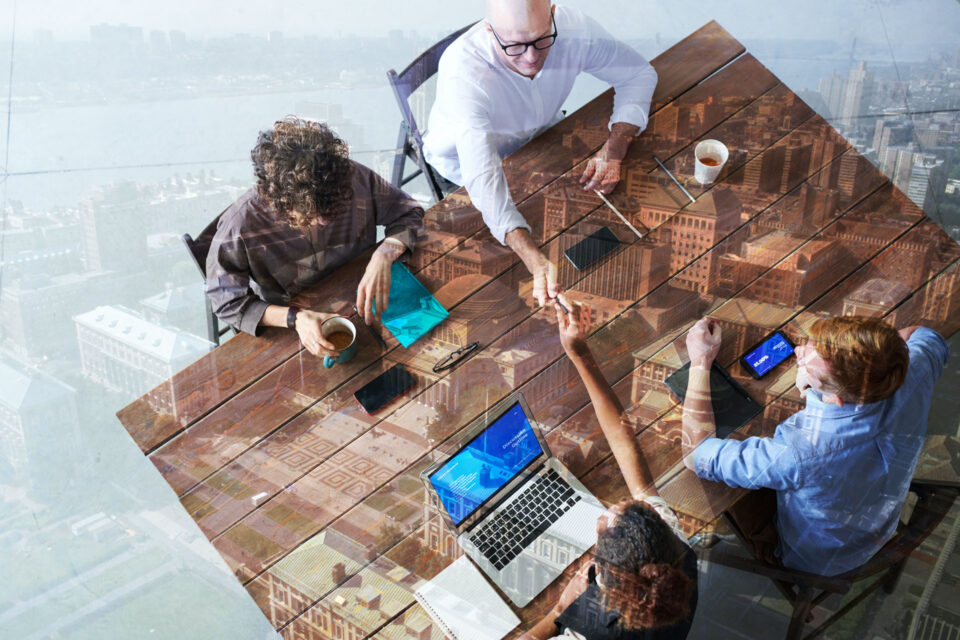
The Data Science Institute’s Seed Funds Program supports new collaborations that will lead to longer term and deeper relationships among faculty in different disciplines across campus. Aimed at advancing research that combines data science expertise with domain expertise, funded research should embody the spirit of the Institute’s mission statement. The following research projects and teams have received 2021 awards.
Racial Politics and Sentiment on Twitter: The Dynamics of Online Emotional Language
Colin Wayne Leach, Psychology, Africana Studies
Courtney Cogburn, Social Work
Sining Chen, Industrial Engineering and Operations Research
Kathleen McKeown, Computer Science
Susan McGregor, Data Science Institute
Social media is a powerful means of individual expression, and collective consolidation, of people’s sentiment about the most important issues in our society. This transdisciplinary project marries the latest advances in computational and statistical techniques of language use over time with social behavioral theories of emotion and stress to examine the temporal dynamics of tweets surrounding police killings of Black people and subsequent protests (e.g., Black Lives Matter).
Harnessing Big Data and ML to Learn Optimal, Individualized Dynamic Treatment Rules to Prevent Opioid Use Disorder Relapse
Sean Luo, Psychiatry
Min Qian, Biostatistics
Kara Rudolph, Epidemiology
Pharmacologic treatment of opioid use disorder (OUD) is complicated by the likely absence of a one-size-fits-all best approach; rather, “optimal” dose and dose adjustment are hypothesized to depend on person-level factors, including factors that change over time, reflecting how well the individual is responding to treatment. This team will use harmonized data from multiple existing clinical trials with natural variability in OUD medication dose adjustments over time to 1) learn optimal dosing strategies, and 2) estimate the extent to which such optimal dosing strategies could reduce risk of treatment drop-out and relapse.
mHealth-Based Data Science Approach to Examine the Association of Minority Stressors with Sleep Health in Black and or Latinx Sexual and Gender Minority Adults
Billy Caceres, Nursing
Ipek Ensari, Data Science Institute
Kasey Jackman, Nursing
This pilot study will use data science techniques to leverage ecological momentary assessment and consumer sleep technology to phenotype sleep health profiles in Black and Latinx sexual and gender minority adults. The investigators will use 30 days of daily electronic diaries and actigraphy to examine the associations of daily exposure to minority stressors (such as experiences of discrimination and anticipated discrimination) with sleep health among Black and Latinx sexual and gender minority adults.
Randomized Methods for High-Throughput Characterization of Tokamak Sensor Streams
James Anderson, Electrical Engineering
Michael Mauel, Applied Physics
Jeffrey Levesque, Applied Physics
Fusion science seeks to advance our fundamental understanding of physics and make plasma fusion viable for applications such as clean energy production. Tokamak fusion reactors generate vast and rich data sets obtained through multiple sensing modalities. The goal of this project is to develop new robust and efficient methods rooted in randomized numerical linear algebra for analyzing and characterizing complex fusion discharge dynamics.
The Columbia-IBM Center for Blockchain and Data Transparency, a joint initiative with the School of Engineering and Applied Sciences, supports novel projects that are exploratory and not yet ready for funding from traditional sources. Successful projects should be able to secure long-term funding from external sources after completion of the seed grants. The following researchers have received 2021 awards.
Converting CO2 to High-Value Polymers Enabled by Data Sharing and Transparency
Luis Campos, Chemistry
The goal for this work is the development of a new model for transparent collaboration between research labs focused on a case study of co-development of new chemical transformations for conversion of CO2 into high-performance materials.
Lightning Network Economics: Cost Minimal Channels and Their Implications for Network Structure
Gur Huberman, Business
This project obtains conditions under which two parties optimally establish a channel in the Lightning Network (a payment protocol that operates on top of a blockchain-based cryptocurrency to increase expediency of transactions), finds explicit formulas for channels’ costs, and derives implications for the network’s structure under cooperation assumptions among small sets of users.
Towards Publicly Available Trusted Carbon Footprints
Christoph Meinrenken, Earth Institute
The breakdown of carbon emissions to most individual products (product carbon footprint, or PCF) is not known, thus making it difficult (i) for consumers to make informed purchasing decisions; and (ii) for companies to reduce their emissions. The goal of this project is to overcome this lack of transparency in PCFs.