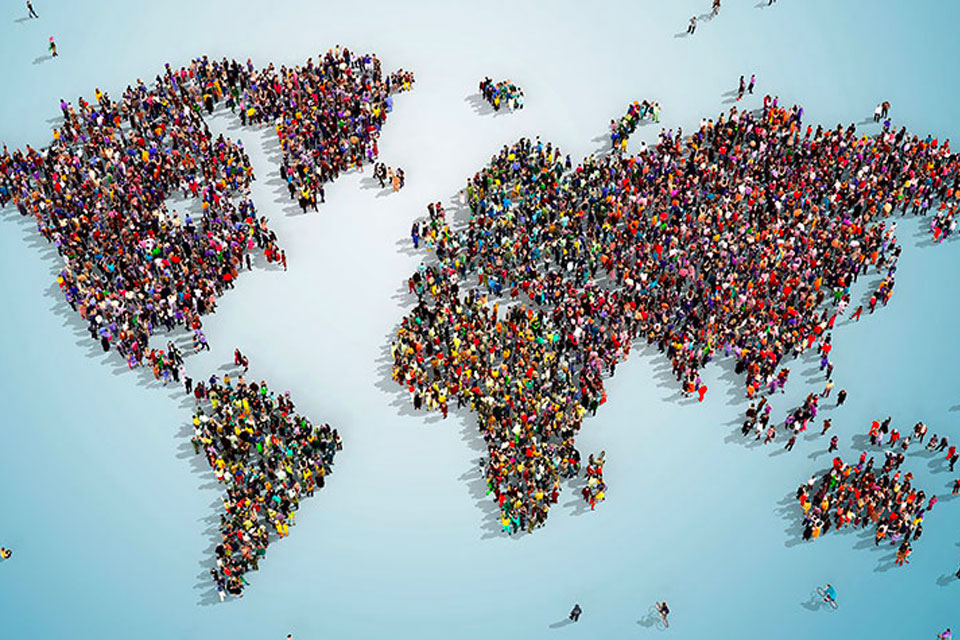
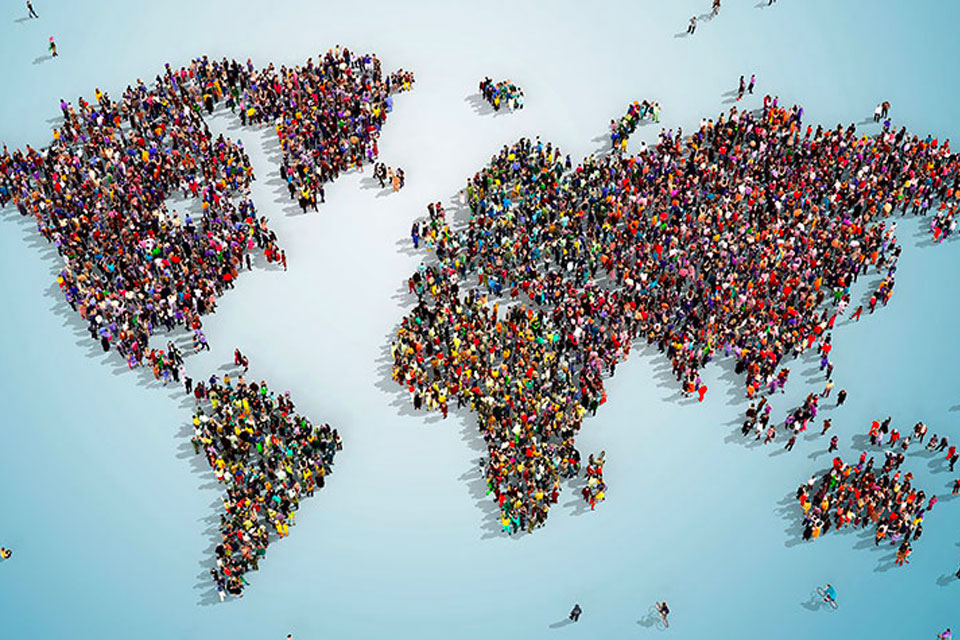
Causal inference leverages artificial intelligence, machine learning, and subject matter expertise to combine multiple, disparate datasets to create “cause and effect” maps known as causal graphs for a variety of scenarios. These graphs help researchers determine possible outcomes in new, never experienced before situations.
The emergent field of causal health sciences performs causal inference modeling on the enormous—but biased and heterogeneous—amounts of observational and experimental health care data. This helps researchers and health care providers determine the outcomes for various medical interventions, saves money, improves quality of care, and accelerates results.
Data Science Institute (DSI) postdoctoral research scientist Adèle H. Ribeiro works with associate professor of computer science Elias Bareinboim in the Causal Artificial Intelligence (CausalAI) Laboratory to develop and apply AI and causal inference methods to benefit public health. She also works with Linda Valeri, an assistant professor of biostatistics, to understand disparities among results that use data sets from different sources and encode differences between populations.
“Extracting causal information from the huge amount of data we have available from a variety of clinical trials and medical records is one of the biggest challenges in health care data science,” Ribeiro said.
Before coming to Columbia, Ribeiro was a postdoctoral fellow in the Laboratory of Genetics and Molecular Cardiology at the Heart Institute at her alma mater, University of Sao Paulo (USP) in Brazil, where she received her doctorate and master’s degree in computer science and her bachelor’s degree in computational and applied mathematics. She also held a doctoral research internship at the Developmental Neuromechanics and Communication Lab at Princeton University.
Today, through the CausalAI Lab at Columbia, Ribeiro develops methods to conduct causal inferences without having to build out an entire graph and working to compute causal effects using smaller subsets of data.
The process involved in any causal inference is often complex and multifaceted as it brings together a wide range of relevant data to model the broader underlying system and identify paths of cause and effect. Once the relationships between observed variables are modeled, a causal graph emerges, illustrating the global view of the underlying system. A causal analysis, conducted based on this view, enables researchers to estimate a potential effect, as well as to distinguish between direct, indirect, and spurious effects.
The process of combining data from different studies requires articulating and then encoding the context, understandings, and assumptions behind each dataset. It also involves incorporating prior knowledge about the relationships between variables (the effect of one variable on another) and the presence of unmeasured confounders (outside variables or factors that may severely distort the associations between measured variables).
“Potential confounding variables are a common problem in observational studies and must be considered and be accounted for in any causal analysis in order to ensure that results are internally and externally valid,” Ribeiro explained.
Close collaboration with subject matter experts is necessary to perform inferences accurately and help explain the disparities between different studies and datasets. Their input helps researchers like Ribeiro build the graphs, and select appropriate graphs from multiple options to develop the “best” model—one that is in accord with both the data and the experts’ understanding of the scenario and context.
In health care, causal inference theory also has practical applications for medical experiment design. By leveraging data from existing studies, causal inference techniques help researchers predict or bound outcomes of interventions and treatments in the target population without having to conduct a new clinical trial. If current knowledge is insufficient, causal inference methods also help determine the design of new experiments.
New techniques being developed in Columbia’s CausalAI Lab would enable researchers to apply causal inference theories and approaches without having to map the relationship between all the variables and construct the full graph. Researchers could fill in parts of the picture, using knowledge from each segment to help clarify the workings of the entire system. “If you know some properties, you can estimate the effect without having to engage with the entire graph,” Ribeiro said.
— Karina Alexanyan, Ph.D.