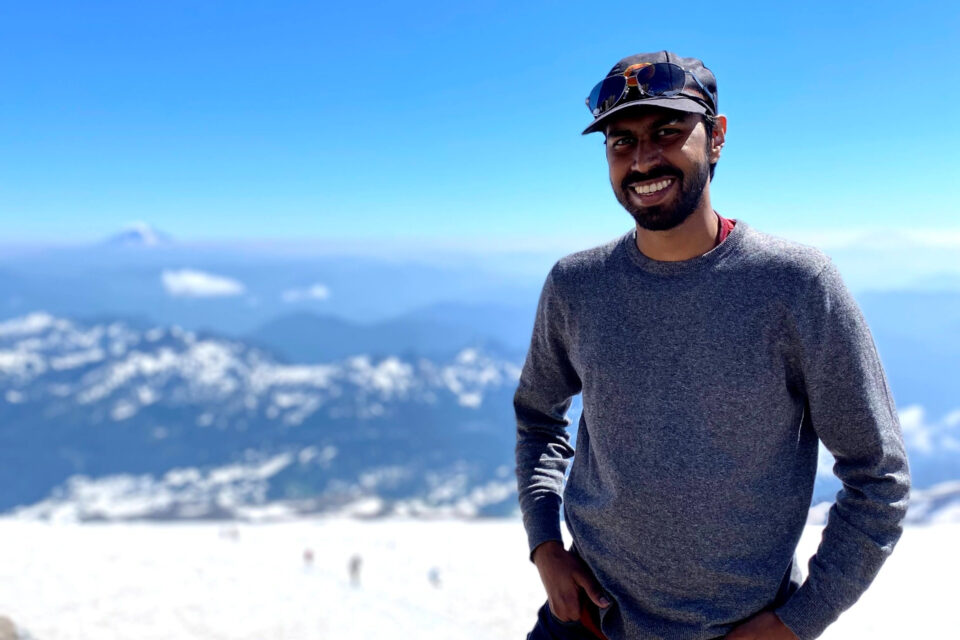
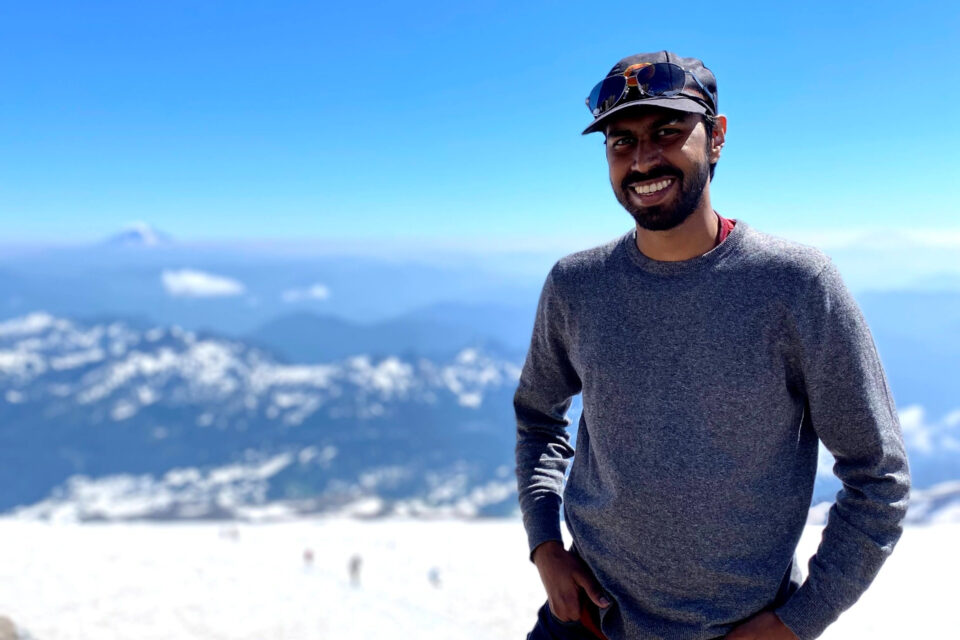
Tarun Devireddy studied systems science at the Indian Institute of Technology Jodhpur and worked for Barclays and Acko General Insurance before enrolling in the M.S. in Data Science program at Columbia University. He has experience in investment banking analytics and growth and product data science, and he recently relocated to Seattle to join Amazon as a data scientist. Here, the 2022 alumnus shares more about his background and his choice to come to Columbia for graduate school.
When did you become interested in data science?
My first experience in data science was with a few friends during my undergrad. We all used to take a lot of flights to go home during every vacation. My friends realized that flight prices are not a monotonically increasing function and that there was scope to build something around it to help ourselves. That project really interested me for practical applications. Prior to that, I was active on Kaggle, but these friends definitely nudged me into fully exploring data science from a business perspective.
Why did you choose to come to Columbia for your M.S. in Data Science?
The data science curriculum at Columbia was what was appealing. Coming with significant work experience, I wanted a program that was a great blend of academics and industry exposure. The curriculum is designed to help understand the breadth of data science and elective options provide you an opportunity to go in-depth into one domain. The opportunity to be in New York and explore and interact with the best minds around campus and in the city was a significant factor in my decision-making process.
What did you enjoy most about living in New York City?
The best thing about New York is its diversity and the perspective that diversity brings. The city is so populated that you can feel like a small piece in the puzzle, but if you find friends or activities that fit well with you, then you can move the whole engine! I lived in Mumbai for a couple of years; New York definitely reminds me of Mumbai. My hometown of Hyderabad is a much slower-paced city in comparison.
How did your undergraduate experience prepare you for our curriculum?
Columbia has a very technical and practical curriculum. The rigor of the coursework requires a great amount of discipline and time management. My undergraduate coursework was predominantly in computer science and mathematics. I believe that helped me a lot. I would say that proficiency in programming, probability and statistics, and calculus are the most important prerequisites to be successful.
Did you complete an internship during the program?
I worked with SwissRe for three months during the summer and with Unilever for the fall and spring semesters. I was given very open-ended problems, which almost seemed like a rabbit hole to explore. I realized the power of communication and asking pointed questions. I experienced the effectiveness of brainstorming to improve crude thoughts, but the sheer nature of explorative work was daunting. I realized the importance of the math behind the algorithms and the methods and that it is not just a programming job. Data science is as much of an art as it is a science.
Tell us about your capstone project.
My capstone project was to build a Semantic Proto-role Labeler (SPRL) using neural networks and natural language processing techniques in partnership with Bloomberg. Semantic Role Labeling (SRL) is the task that answers the question of “who did what to whom, when, where, and how” in a sentence. As an extension to the SRL, the concept of SPRL is to build confidence in the SRL tags. They break up each role into a set of features and assign scores to each of those. This can further fuel the understanding of how the argument is involved in the event and can help in many tasks, including the creation and interpretation of knowledge graphs, understanding the impact of news articles on financial stocks, and similar tasks.
What were your key takeaways from your Columbia data science experience?
The three most important things I believe are the most fulfilling to me are: a) a great community of like-minded people passionate and deeply interested in the data science field; b) practical opportunity through internships, coursework, and projects in terms of multi-tasking, managing stress, and structuring vague problem statements; and c) being able to explore the breadth of data science and find the niche that actually interests me, something I’d love to do for a very long time.
This interview has been edited and condensed for clarity.