Uncovering Neglected Stories of Enslavement with Machine Learning Tools
January 6, 2022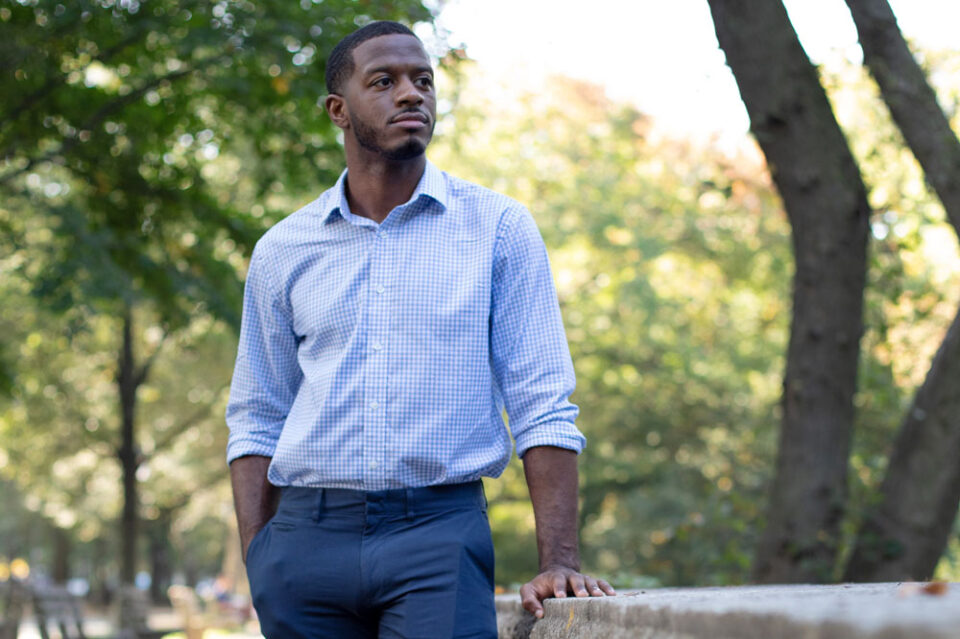
Historian Abraham Liddell studies the interpersonal networks of free and enslaved Africans in 16th and 17th century Spain, Portugal, and Latin America, but he was driven to data science by his frustration with how difficult it is to find records on marginalized groups during the period.
While the trafficking of African humans powered much of the early modern Atlantic economy, first person narratives of marginalized groups from the era are virtually nonexistent. Researchers typically extract relevant data from other records in which free and enslaved Africans and their descendants may have a voice, including court cases or ecclesiastical records.
“Finding this information becomes a specialized skill set. You need to understand archival structure, historical record keeping, etc.,” Liddell said. “I remember thinking it would be really great if one could train a [machine learning] model to find the people I’m looking for in the records for me.”
He decided to merge the humanities, social sciences, and data science to build what will be one of the largest singular databases on free and enslaved Africans of the early modern Atlantic era and, eventually, enable others to interrogate the data and ask their own questions.
“It’s what I’ve always wanted to do—to pull marginalized people from the records so that we can look at them, see their histories, see what we can learn about them and their lives,” Liddell said. “It makes me happy because that means that some grad student, a few years from now, will be able to use all of this data and write a dissertation out of it in ways that I couldn’t.”
Liddell started this work with transcribed records from Vanderbilt University’s Slave Society Digital Archive (SSDA), which is an extensive collection of serial records documenting the history of Africans in the Atlantic world. He collaborated with data scientists to develop a natural language processing (NLP) pipeline to extract pertinent information, including names, dates, locations, and relationships, from these transcriptions as part of his doctoral research in Latin American history.
Today, as a postdoctoral research scholar at the Data Science Institute at Columbia University, Liddell collaborates with Augustin Chaintreau and Christopher Brown to develop tools that will identify relevant information and, ultimately, to better understand the structure and evolution of interpersonal and community relationships of Cubans of African descent. They will tackle the project in three parts:
While each step in the process will have its challenges, the first step is particularly laborious since it requires poring over thousands of handwritten pages, which are difficult to decipher and often have inconsistent spelling and writing styles even within the same document. Such inconsistencies make training ML models and automated optical character readers rather arduous and problematic, according to Liddell.
Once the transcriptions are complete and the relevant data is extracted, Liddell will be able to focus on what he considers the most interesting part—developing a framework for data analysis. Ideally, the model will reliably predict or infer relationships, even if they aren’t explicitly articulated in the original text. He should also be able to reconstruct communities, see how they interact, where they overlap, how they change, and in what ways.
“There are all sorts of interesting insights about relationships and behaviors that this dataset can generate,” Liddell noted. “How often do they intermarry and have children with each other? What groups appear side by side and form clusters of communities with one another? Do you see evidence of relationships between these groups in the ‘new world’ that reflect how they interacted in Africa?”
— Karina Alexanyan, Ph.D. and Shane Tan