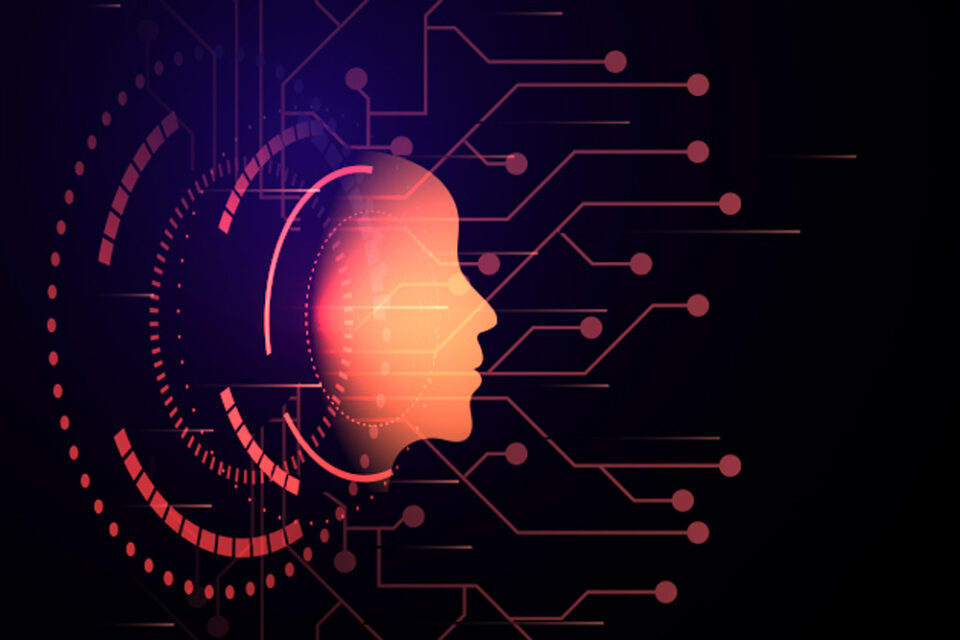
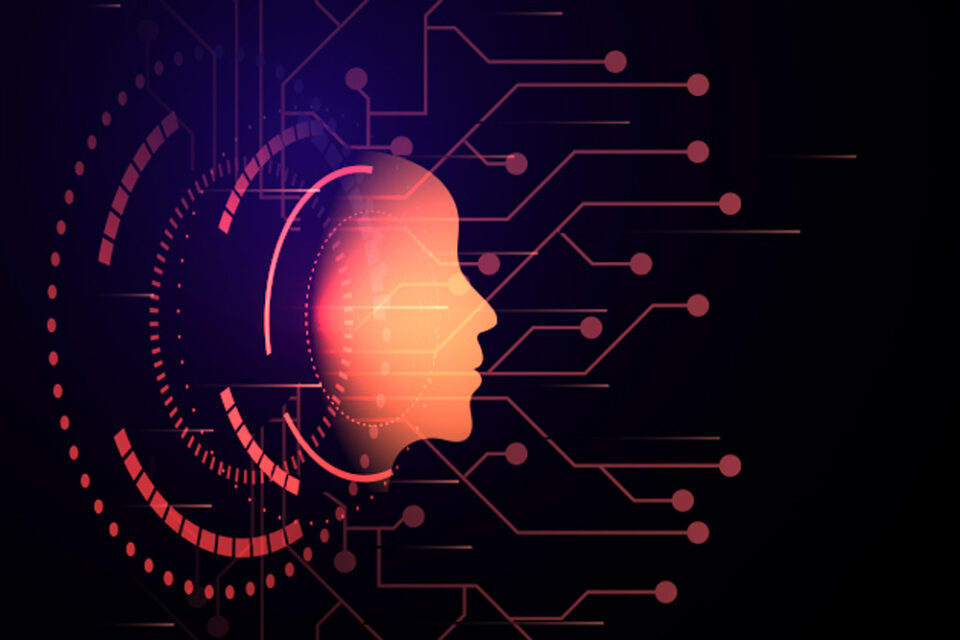
Working at the intersection of data science, computer science, and cognitive science, Zenna Tavares explores how humans reason and learn—and builds computational systems to reproduce these processes.
“I’m trying to better understand natural intelligence, reasoning, and inference, while also building tools that emulate and model human cognition,“ Tavares said. “I see these areas of my work as synergistic and mutually reinforcing.”
As a Columbia University Data Science Institute associate research scientist and Zuckerman Mind Brain Behavior Institute innovation scholar, Tavares develops frameworks that allow domain experts to encode their knowledge and observable data and ask virtually any question about the model, including predictions and projections into the future and the past.
Tavares leverages two types of reasoning—probabilistic and causal. The former involves the way we calculate probabilities in uncertain situations; the latter involves how we assess cause and effect, including our ability to think through counterfactuals. For example, he may use infection rates to infer the transmissibility of a disease, and then ask whether the rates would have been lower under different public policies.
Most machine learning (ML) models are “bottom up” and trained for pattern recognition with massive data sets. Tavares describes his approach as more “top down”—he starts with a causal mechanistic model of how elements in the environment relate and interact. “It’s not a new idea,” he noted. “What is new is that now we can build computer systems that can realize this idea. We can use these very general languages and representations, such as causal probabilistic programming languages, to encode knowledge and data and semi-automatically derive inferences.”
While Tavares finds building models that reflect causal relationships in the data to be one of the most challenging aspects of his work, he considers the ability to ask ‘what if?’ questions necessary. His goal: “To build systems that can assess and synthesize causally fair, including counterfactually fair, ML models for specific scenarios, such as job applications, financial assistance, etc.”
Tavares received his Ph.D. in cognitive science and statistics from the Massachusetts Institute of Technology and was a postdoctoral scholar with Armando Solar Lezama at the Computer Science Artificial Intelligence Lab. He conducted research in program synthesis to bring more automated reasoning and learning to programming while using code, programming, and modeling to improve learning systems. He also collaborated with Joshua Tenenbaum‘s computational cognitive science group to use mathematical modeling, computer simulation, and behavioral experiments to better understand human learning, reasoning, and inference from a computational perspective.
At Columbia, Tavares works on causal interpretations of fairness, trustworthiness, and explainability to address core issues in artificial intelligence (AI) decision making. He will expand his team this year and plans to host a debate series on cognitive science, AI, and data science.
“Fundamentally, the thing that interests me is knowledge and reasoning,” Tavares said. “I think we’ve just started to scratch the surface of understanding what that is and how that works.”
— Karina Alexanyan, Ph.D.