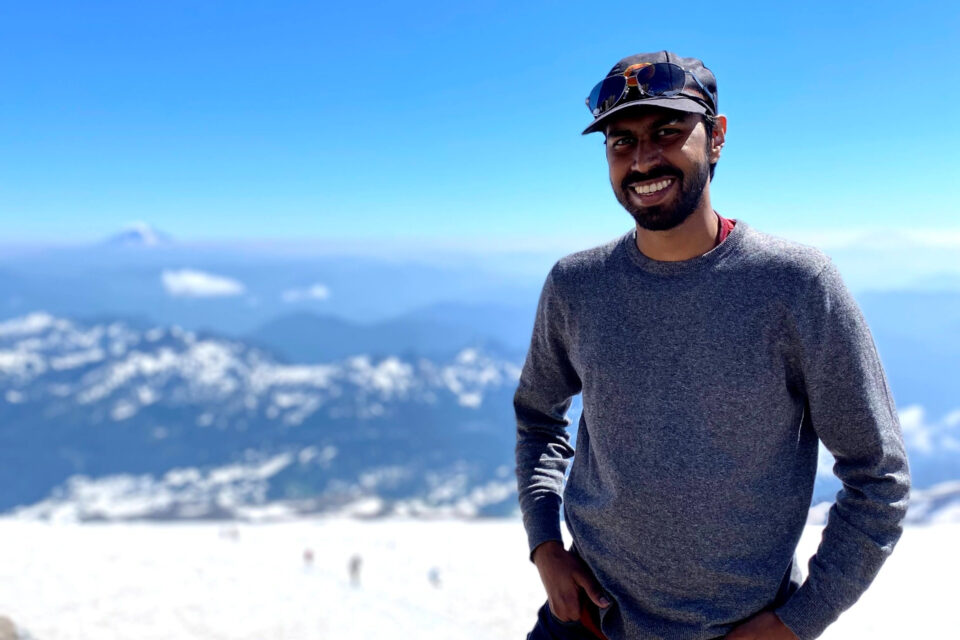
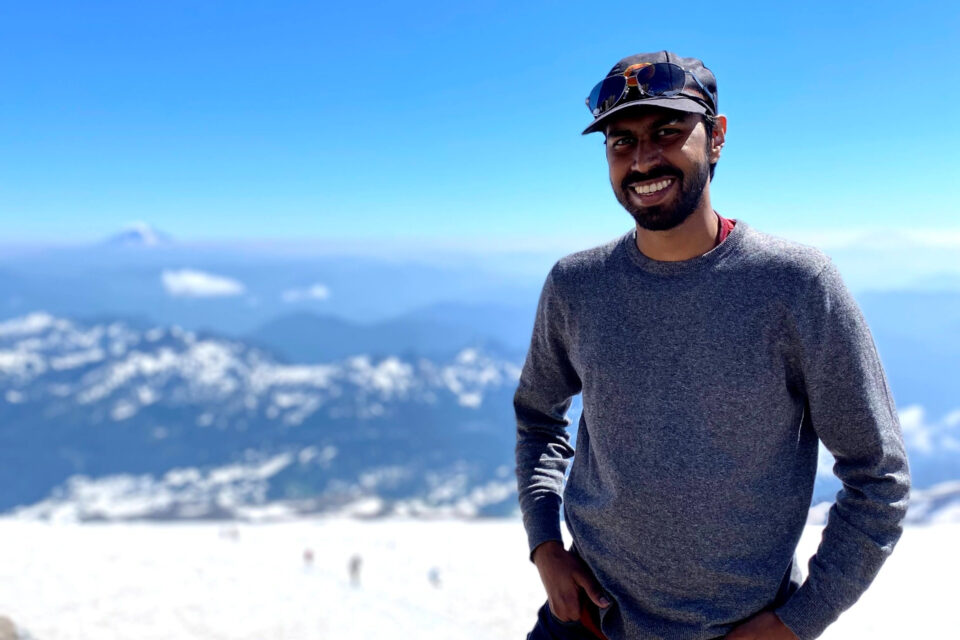
From raw material procurement to the delivery of finished products, supply chain management is pivotal for the success of retail businesses. To keep pace with consumer habits both in-store and online, companies are increasingly relying on data to predict demand, improve inventory, optimize transportation routes, and ensure quality control of goods and services shipped around the world.
Amazon is a notable example of this transformative impact. Through its extensive use of advanced analytics and algorithms, the e-commerce giant can process and evaluate massive volumes of data in real-time, showcasing how data science can redefine the boundaries of supply chain efficiency.
In his final semester at Columbia, Tarun Devireddy (M.S. Data Science ’22) received the offer to move to Seattle and join Amazon’s growing data science team. His focus is on building machine learning solutions to improve shipping and minimize damages to products. With “customer obsession rather than competitor focus” as one of Amazon’s core values, Devireddy’s team helps ensure positive experiences, from international warehouses to the customer’s front door.
Before enrolling in the MSDS program, Devireddy studied Systems Science at the Indian Institute of Technology Jodhpur and held positions at Barclays and Acko General Insurance. Coming to campus with extensive work experience in business analytics and product, he was enticed by Columbia’s commitment to blend rigorous coursework with real-world training.
The Data Science Institute talks to Devireddy about his experience in the MSDS program, and how it set him on the path to grow his career in the e-commerce sector.
This alumni interview series is part of our year-long celebration to mark the 10 Year Anniversary of the M.S. in Data Science Program. Follow more updates with #MSDS10.
Since leaving the M.S. in Data Science Program, you’ve started an exciting position as a Data Scientist at Amazon. Tell us about your experience in the tech industry. What are some data science projects you’re working on in the tech space?
I work with an amazing team of data and applied scientists called QuBIT Midas. They are always willing to share knowledge and help us raise the standards of models and analyses we develop. Alongside them, I learn something new every week! Working at Amazon has been particularly interesting because of the scale of data. Understanding the trade-offs across the different options available to handle large amounts of data, training models on these large datasets, and making efficient inference pipelines – all of that has been an enriching learning experience. We rarely get such end-to-end experience in academia.
Importantly, I have learned that data science problems are not only about developing the right technical and scientific solution, but also significantly more about the business impact, interpretability, and guardrails.
I’m currently working on the problem of identifying appropriate packaging requirements for the items that we ship to customers, in order to avoid damages while also minimizing the packaging costs. From the technical standpoint, the problem involves building a multi-modal architecture in a supervised learning setting, and uses tabular, textual, and image data. I’ve also worked on a couple of forecasting problems related to customer return volumes in order to guide labor planning decisions in our returns-handling network.
What lessons from the M.S. in Data Science program are you using in your career? What are some practical applications that you are using every day?
Every concept taught in the machine learning courses—ranging from supervised/unsupervised learning to understanding optimization functions—are used in almost every project within my team. We are constantly experimenting with different algorithms and benchmarking them against each other to arrive at the optimal solutions for Amazon’s customers. We employ various experimental frameworks to measure the impact of our solutions. The Statistical Inference course in the MSDS curriculum covers the concepts on hypothesis testing in depth, and I refer to them frequently.
During my summer internship and Capstone project, I worked on multi-task learning and transfer learning approaches. I currently use both of these techniques in my most recent packaging-optimization project. At Amazon, we have numerous models that are built and trained on millions of data points, and using novel approaches to fine-tune them for individual use cases is a very important and practical application in the industry.
Can you share how the MSDS program helped you prepare for a career?
Firstly, the MSDS program placed a lot of emphasis on getting our hands dirty with data and practicing with many of the traditional and evolving analytical solutions used by industry. To add to that, I also received the opportunity to work with multiple companies throughout the duration of the program, where I learned how to apply these concepts practically.
Secondly, my summer internship at SwissRe was to build a custom entity recognizer, which was right after taking the Natural Language Processing (NLP) course. I now use a lot of concepts from the course and my internship while working with textual data related to product titles and descriptions for the packaging project. My fall internship at Unilever also provided me with an opportunity to navigate ambiguity across the business aspects of problems, which is a significant part of scoping any industry project.
Lastly, the MSDS program provides a great alumni network which is highly knowledgeable and accessible. I was part of the Amazon Machine Learning Conference last winter where I ran into some alumni of the program and had thought-provoking discussions about entrepreneurship and data science for environmental and social impact.
What was the last thing you used ChatGPT for?
I’ve used ChatGPT a bunch of times recently. One of my favorite times was when I asked it to plan my road trip over a summer weekend around Seattle. The most recent instance I’ve used it for was to coin a name for my project. ChatGPT was able to give me a plethora of options to pick from as I kept giving it additional instructions in order to improve the recommendations.