Columbia Data Scientists Discuss the Nature of Fairness at Data Science Day 2024
April 23, 2024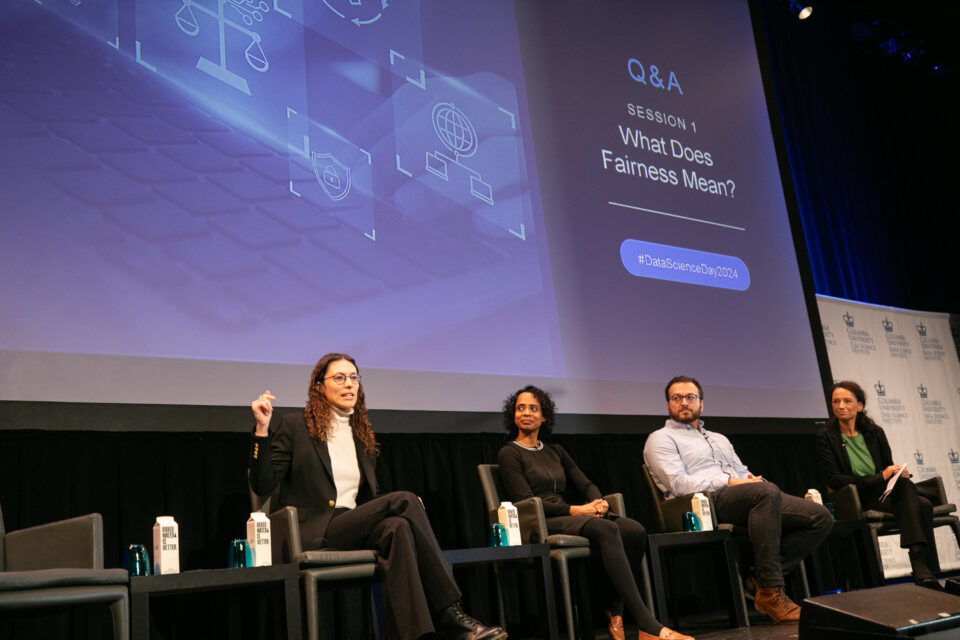
Columbia’s Data Science Institute gathers researchers from many disciplines in search of pragmatic ways to address some of modern society’s most pressing issues, including climate change and sustainability, human health, and the future use of technology. Fairness is a pressing issue throughout: How can we be sure our data is representative? How can we understand the best ways it can be used? What do innovations like Artificial Intelligence tell us about the fairness of our society itself?
Katja Maria Vogt, a Professor of Philosophy and PI on the Values Lab, who moderated the session, opened by asking not what fairness meant but how fairness came to take the spotlight in cultural conversations about ethical AI. She posited that “fairness” is often used in place of “justice” is as a way to ground discussion and sidestep big philosophical questions, a hypothesis drawn from a John Walds paper that posits that fairness is considered more of a workable notion than justice.
Vogt suggested that maybe there is no way around the big picture questions and interdisciplinary contexts like the Data Science Institute play a crucial role in helping society pose them.
Nothing illuminates the problem of fairness like the pricing and access to goods and services. There is the discriminatory pricing of the so-called “pink tax,” a term for the generally higher prices charged for products marketed to women, or in the loan rates that favor one race or gender over another. Yet there are also price discriminations designed for potentially beneficial social outcomes, like college tuition breaks for certain categories of students. Looking at online vehicle sharing, Prof. Elmachtoub addressed two different types of fairness, price affordability and vehicle availability.
Even with no resource constraints, he said, “it’s impossible to achieve perfect fairness in price and access at the same time, except for very weird instances.” When prices are the same to all, the lower income group tends to be priced out over the long run. When access is stressed, the supply/demand balance may enter dysfunction. “Sometimes we have good intentions with our fairness, but if we don’t consider the operations of our system, it can have lose/lose effects for everybody,” he said.
Adam Elmachtoub is Associate Professor in the Department of Industrial Engineering and Operations Research at Columbia Engineering
Assistant Professor Shalmali Joshi and colleagues analyzed psychosis patients in Medicaid to evaluate the feasibility of training AI models to predict which of those patients may go on to develop schizophrenia. In broader literature, it is well known that many factors like social determinants of health, healthcare-seeking patterns, and implicit clinician bias, among others, contribute to disparities in mental health diagnosis. Simultaneously, it is epidemiologically known that African Americans, for example, are 2.4 times more likely to be diagnosed with schizophrenia compared to their White counterparts, are more often misdiagnosed, and are given antipsychotic medications at a higher rate than white patients.
Joshi found that one of their AI models for predicting new-onset schizophrenia among psychosis patients showed lower sensitivity for Black patients compared to White patients. Joshi set out to quantify how each of broader known disparities contributes to the unfair predictions of the AI model. Joshi identified that in their data, these broader disparities compounded together in different proportions to contribute to the disparate sensitivity of the AI model. Joshi’s method quantifies the extent to which each factor contributes to the sensitivity gap.
Her data analysis showed that our AI models are trained from data which is “the exhaust of a very complex ecosystem, (which) we need to start characterizing and modeling,” to understand broader disparities and how they contribute to unfairness in AI predictions. Artificial Intelligence can be invaluable in this kind of large-scale data analysis, since “once we measure these sources of disparities we can look at targeted policies that can help change, for example, healthcare utilization patterns.”
Shalmali Joshi is Assistant Professor in the Department of Biomedical Informatics at Vagelos College of Physicians and Surgeons
AI can find and leverage patterns in large data sets, enabling new insights and speeding action. There can be a cost, however: Different types of AI models, trained on similar data and achieving similarly efficient business outcomes, can still vary widely in how much they discriminate against different groups of people. In her talk, Professor Black showed how slight changes in one just AI model achieved markedly higher and lower levels of discrimination.
This has powerful implications for areas like housing, credit, and employment, where models are commonly used and discrimination is prohibited by law. ”It makes little sense to say that an algorithm is necessary or justified if it displays disparate impact,” she said. In the future, she said, companies may be forced to show they did a proactive search for a less discriminatory model.
“There is a proactive duty to search for less discriminatory algorithms,” she said. Current law, however, prohibits the use of certain types of attribute information, like race or gender in the three greatest use cases. “How do we navigate this conundrum?” she said. It is the goal of her future research.
Emily Black is Assistant Professor in the Department of Computer Science at Barnard College