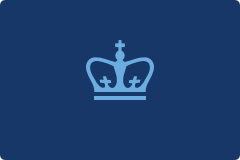
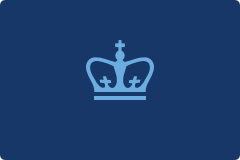
Our third-annual Data Science Day will feature lightning talks by Columbia professors using data science to strengthen computer security, improve health care, and study the implications of new business platforms and our growing sharing ecoomy.
The second part of the day will feature live demos and opportunities to speak with students and researchers about current projects. Alfred Spector, chief technology officer at the financial services firm Two Sigma, will give the keynote: “Opportunities and Perils in Data Science.” The event will conclude with a networking reception.
Summaries of the lightning talks are listed below.
DATA SCIENCE DAY | Columbia University | April 5, 2017 | 8 am to 5:30 pm
OUR CONNECTED WORLD
Suman Jana: Security and privacy in a hyper-connected world
From smartphones to cameras, most modern computing devices are connected to the Internet, and fitted with multiple high-bandwidth sensors that collect potentially sensitive data about their user’s environment. This abundance of contextual information allows app developers to improve the technology, but poses significant security and privacy risks to individuals. Making matters worse, most devices support untrusted third-party applications downloaded through online software markets i.e. “app stores”. One such app, https://funstore.io/,lets consumers download and execute thousands of untrusted third-party Internet-of-Things (IoT) applications on different “smart” devices. In this talk, I will present an overview of emerging security and privacy challenges and ways that we might address them.
Hollie Russon Gilman: How Civic Tech Feeds Urban Innovation
New technologies are increasingly helping ordinary people make their cities more innovative and responsive to public needs. By strengthening democratic participation, civic technology, or civic tech, offers new ways of giving citizens access to government decision making. In this talk, I will discuss three ways that civic tech is fostering collaborative governance in U.S. cities—through innovation units, open data and crowdfunding. I will explain what appears to be working and why, and how greater digital literacy and Internet access can amplify these trends.
Susan McGregor: Privacy Protections Make Us More Secure
Privacy and security are often presented as mutually exclusive, with government agencies framing accessible encryption and other privacy-preserving technologies as national security threats. Yet in a world of ubiquitous networked computing, these technologies are essential to protecting basic constitutional and human rights, as well as America’s economic and political integrity. Journalists depend on these technologies to protect sources and colleagues, so that they can report freely and independently on the news of the day. I will discuss the myriad ways in which stronger privacy also strengthens security for journalists, academics and Americans more broadly.
DATA SCIENCE APPLICATIONS
Mingoo Seok and Stefano Fusi: Brain-Inspired Learning Machines
Deep neural networks, or networks with multiple hidden layers, are revolutionizing machine learning and cognitive computing with their ability to classify images, videos and speech as rapidly and accurately as humans. The algorithms for training a deep neural network, however, are complex and require huge computational resources to tune the millions of parameters used in the training process. One way to minimize computing time is to limit each parameter’s precision from 32 bits to 1 bit. Though this reduces parameter precision, classification performance remains strong, theoretical studies show. Looking to the brain for inspiration, we are developing new methods that build on this approach.
Andreas Mueller: The Rise of Open Source Software for Data Science
The recent surge in data science applications was enabled not only by the availability of data, but also free and open software tools for processing and analyzing data. Open source projects, primarily in the R and Python programming languages, have been the backbone of most recent work in data science. The scikit-learn project in particular has become the go-to solution for machine learning algorithms in many areas. I will discuss the scikit-learn library, its scope and development, and raise questions about current model of open source tools based on volunteer labor.
Andrew Gelman: From Public Opinion to Probability Theory and Back
What does the theory of stochastic processes have to do with public opinion? It goes like this. We learn about opinion through surveys. Most people won’t respond to a survey so we need to adjust our sample to match the population. To do this right, we need to adjust for many variables. This adjustment requires models with many parameters. To fit such a model involves exploring the needle of good fit within the haystack of possible parameter values. This exploration is performed most efficiently using programs such as Stan that use gradients and other measures of the geometry of the zone of parameter space that is consistent with data and prior information. Advanced mathematics is required to develop the fitting algorithms that use gradient information. Also the fitting should be fast: our models are all wrong, so we want to be able to fit lots of models and use graphical tools to explore the fit to data.
PATIENT-DRIVEN HEALTH CARE
Ken Cheung: Using Patient-Generated Data to Find the Best Health App
Adaptive design is a methodology used in clinical trials that helps researchers compare different drug treatments based on interim observations of trial participants. Its interactive nature also makes it a good framework for evaluating dynamic health apps which evolve quickly over time. In this talk, I will show how adaptive design can be applied to app monitoring and recommendation within an ecosystem of health apps, and introduce SMART-AR, a framework for analyzing data in this ecosystem. I will discuss the analytics we are developing for Android mental health apps in a collaboration between Columbia and Northwestern universities.
Kenrick Dwain Cato: Identifying At-Risk Patients from Nursing Notes
More than 200,000 patients die in U.S. hospitals each year from cardiac arrest, and more than 130,000 patients die of sepsis, a deadly immune response to bacterial infection. Many patient deaths could be prevented if the warning signs could be caught sooner. Our research suggests that the notes taken by nurses periodically describing their patient’s condition can provide powerful clues as to which patients need extra oversight. I will discuss a project that I am leading at Columbia, Communicating Narrative Concerns Entered by RNs (CONCERN), in partnership with several other teaching hospitals, to automate the analysis of nursing notes in patient electronic health records. Our aim is to design and evaluate clinical decision support system to identify words in nursing notes that best predict a life-threatening health problem. This project is scheduled to begin in June.
Noemie Elhadad: Citizen Endo: (Citizen + Data) Science for Endometriosis
Ten percent of women are thought to suffer from endometriosis, a chronic condition associated with infertility and painful menstrual cycles. Despite its prevalence, we still know surprisingly little about how endometriosis develops, evolves, and how to best treat it. To learn more, my students and I recently launched Citizen Endo, a crowdsourcing project that gathers data directly from endometriosis patients via a mobile phone app we developed. While most research so far has focused on surgical treatment of endometriosis, Citizen Endo, which now has more than 1,500 participants, aims to gain a more systemic understanding by analyzing the day-to-day experiences of women living with the condition.
SHARING ECONOMY
Costis Maglaras:How Ride-hailing Platforms Optimize Performance
Ride-hailing platforms such as Uber and Lyft match passenger demand to driver supply over a geographic network. They have at their disposal several control capabilities, such as how to prioritize passengers based on their destinations; when and which passenger requests to reject; and when and where to direct idling drivers on the network. I will discuss the impact of these type of matching and capacity optimization decisions to the network’s performance, and show that that they lead to significant improvements in most scenarios and work particularly well during the morning and evening rush hour when passenger flows across the network are most unbalanced.
Sharon Di: A New Perspective for Shared Mobility Systems
Ride-sharing and other shared mobility systems promise to reduce traffic, energy consumption, and the amount of time and money we spend traveling. Many of these benefits, however, remain unproven. If policymakers are to develop effective ride-sharing policies, they need to know how travelers are redistributed while using the system. In this talk I will discuss new research findings on ride-sharing that can be generalized to other shared mobility systems. This work is based on a mathematical model for predicting how travelers move across a network of roads. Early results suggest that high-occupancy toll lanes, proposed as one way to encourage carpooling and ease congestion, may be ineffective if poorly planned.
Eric Talley: A Machine Learning Classifier for Fiduciary Duty Waivers
The SEC requires publicly-traded companies to periodically disclose important information to the public, but predicting how and where companies will file these disclosures is extremely difficult. I will discuss a supervised learning strategy to analyze one type of SEC disclosure filing—fiduciary duty waivers, signed by company officers and directors waiving their fiduciary duties to shareholders. Using a lawyer-coded training set of these waivers, we calibrated a predictive classifier and extended it to the entire SEC database. The results of simulated out-of-sample Monte Carlo are strong using conventional evaluation criteria. We find mildly positive effects on securities market prices, suggesting that the waiving of fiduciary duty by company officers and directors is not received as a negative signal by investors and capital market participants.
Further reading:
Data Science Day at Columbia 2016