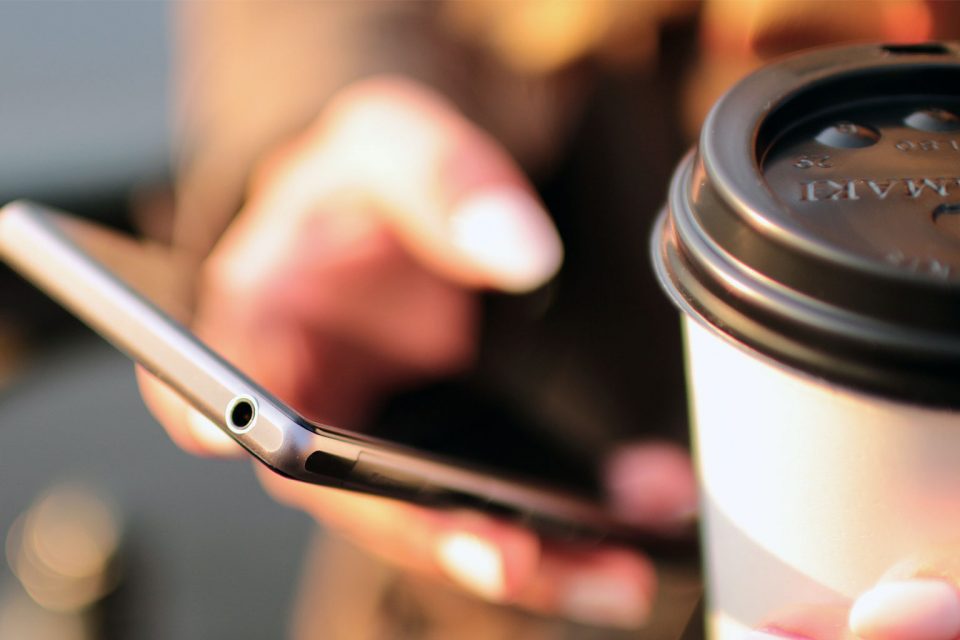
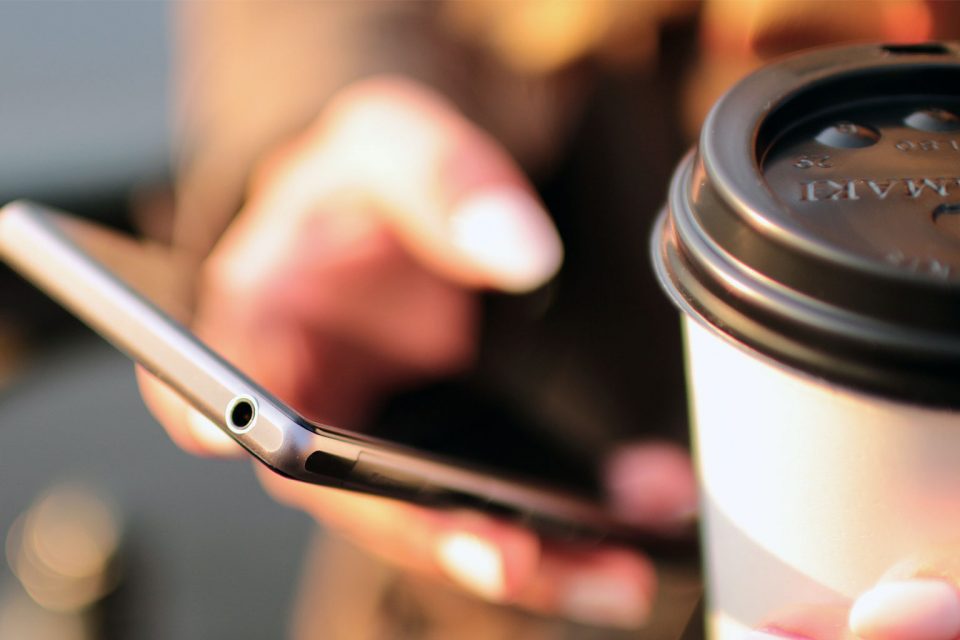
Online searches for information, from Google searches to social media inquiries, threads, and discussions, generate an abundance of data. This data offers researchers a real-time window into people’s needs and pressing concerns. Natural language processing (NLP) methods enable researchers to discern rising interests in specific topics and assess the needs of a population much more quickly than traditional survey methods.
Adam Poliak, a Roman Family Teaching and Research Fellow in Computer Science at Barnard College and Data Science Institute member, uses “text as data” by applying NLP techniques to online conversations for insights into emergent concerns and topics. He collects and analyzes large quantities of text from social media, and uses both “top down” and “bottom up” approaches—creating lists of terms to analyze, or letting lists be generated by high frequency terms as they appear.
“We may start with an assumption of terms that we’re looking for, and then sometime during the data exploration phase, discover terms and phrases that we didn’t know of apriori that are beneficial in telling the story or open our eyes to another phenomenon,” Poliak said. “It’s an iterative process. It seems straight forward in retrospect, but it requires a lot of iteration and getting your hands dirty with the data.”
Poliak received his doctorate in computer science from Johns Hopkins University, where he was affiliated with the Center for Language and Speech Processing and worked on a project supported by DARPA’s Low Resource Languages for Emergent Incidents (LORELEI) program to develop NLP models using Twitter data to detect emergency needs during disaster events.
Poliak’s work with Twitter data revealed issues related to biases in the data that led to biases in his models. This prompted an exploration of data bias, and spurred Poliak to develop advanced methods to help models overcome biases, such as diagnostic tests that evaluate the reasoning capabilities of contemporary NLP models. He also used machine learning to reveal how some NLP models might “cheat” by taking short cuts that researchers don’t know are there, and surfaced how models are doing things that are different from what was expected. “Understanding where and why NLP systems fail is vital to making NLP systems that are more fair, equitable, and accessible. This can prevent systems deployed in the real world from further perpetuating damaging biases,” Poliak explains
At Columbia, Poliak has used online search data and social media analysis to examine a range of topics, from a rise in acute anxiety during the early stages of the COVID-19 pandemic, to a federally funded project examining consumer conversations, especially health claims, around new e-cigarette tobacco products that are popular on social media, to research around public interest in police reform.
His police reform research, published in 2020, revealed that in the 41 days following George Floyd’s murder, there was a 150-fold increase in Google searches for specific policing-related queries. The data strongly suggests that the public has a growing interest in police reform, and offers interesting findings on how searches differed by state. Some states searched for police “training” more than any other reform topic, while others searched more for police “union(s)”. Differing search trends in different states highlight residents’ varying needs, and can help local policymakers use state-specific trends to identify the types of reforms that are best suited to their constituents.
Poliak also collaborates with Caitlin Dreisbach, who is a DSI postdoctoral research scientist, on a series of projects that use social media to explore the impacts of COVID-19 on frontline workers and healthcare providers. Their research examines health care-related coronavirus conversations on Twitter and Reddit by looking at the personal health narratives that surface around clinical care, from patients as well as from health care workers and related communities. Their findings will be compared with “pre-COVID” research on related topics. The goal is to better understand what’s working, what isn’t, and for whom.
“What I really like about this work is that it requires partnering with subject matter experts,” Poliak explains. “Working on computer science at a liberal arts college like Barnard enables us to address the limits of our methods, and access insights and analysis from domain experts. We need computer science tools to put structure on large amounts of text, and we need domain experts to help us ask the right questions and understand the findings.”
— Karina Alexanyan, Ph.D.