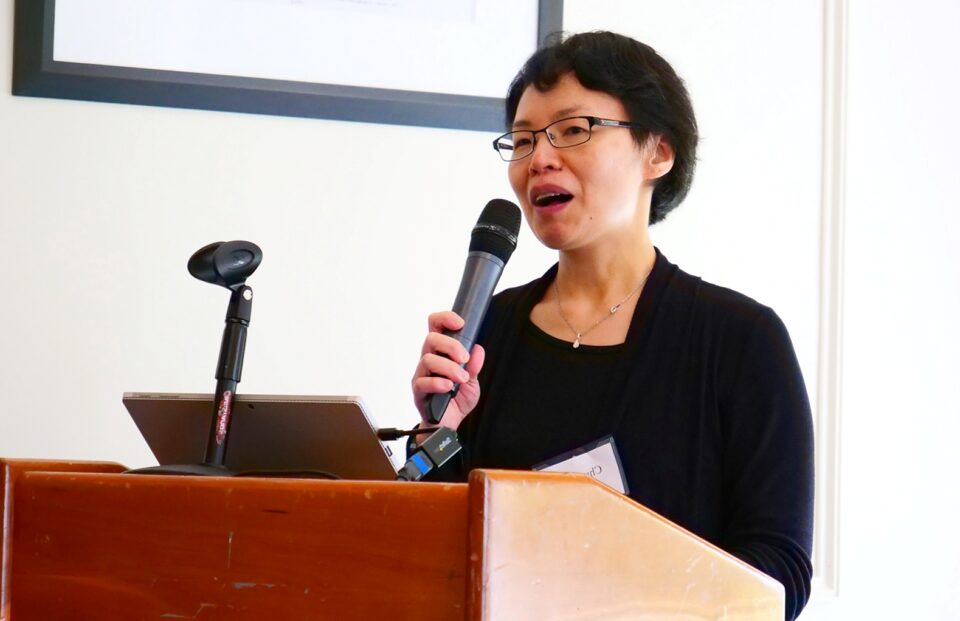
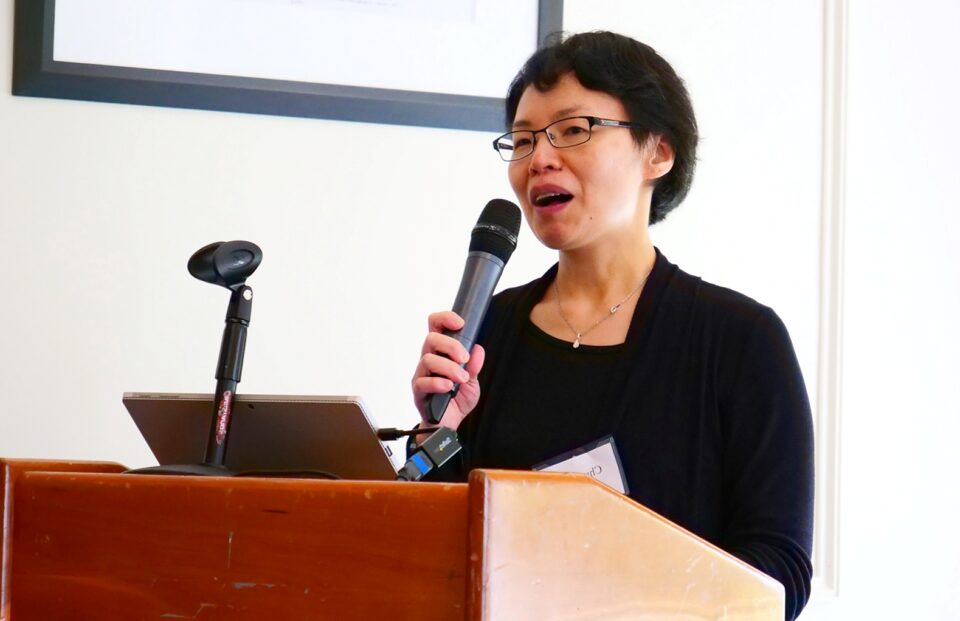
Columbia University biomedical informatics professor Chunhua Weng uses data science to analyze and make sense of electronic health data for knowledge discovery and application. One of her goals is to minimize biases in design and optimize the generalizability of clinical evidence.
Weng’s team at Columbia’s Vagelos College of Physicians and Surgeons analyzes published randomized controlled trial research to evaluate the quality of the evidence and determine “which evidence is good, which evidence is bad, which evidence is biased, and which is underpowered, not patient-centered, or involves controversies or has conflicting evidence.”
Weng began this work by building algorithmic systems to automate processes and analyze data at scale, but she realized how important it was to recognize and identify elements of bias in clinical research populations. “Most research is done on healthy, young, male participants. Our evidence for children, older patients, pregnant women, or sick people with multiple comorbidities is still very scarce,” she said.
Once attuned to the algorithmic bias, Weng began to see how it may manifest in other areas. For example, electronic health records contain more data on sick patients than healthy patients and more information on patients who speak the same language as the doctors. Such an imbalance may skew the results of the machine learning algorithms and risk modeling built with that data. The conclusions, generalizations, and decisions made on the basis of these results may be less accurate and effective.
To tackle this challenge, metadata is extracted from the research, including which health outcomes are being studied, sample characteristics, sample size, and how representative the sample is of the broader patient population. Automated methods extract relevant information from the literature, and models are built to detect and flag bias, such as a study based on a small, non-representative sample.
Weng, who is an affiliated member of the Data Science Institute’s Health Analytics center, has mentored and worked with more than a dozen data science and statistics master’s degree students on a range of data sources since 2019. Some of these students have gone on to pursue doctorates in biomedical informatics at Columbia, information science at Cornell University, biostatistics at the University of Michigan at Ann Arbor, computer science at Hong Kong University, and industrial engineering at Georgia Tech.
Ali Turfah worked with Weng for about 10 months in 2020 and 2021 while completing an M.A. in statistics at Columbia. He believes the research experience was an advantage during the University of Michigan’s biostatistics Ph.D. admissions process thanks to the concrete research examples he could offer in his statement of purpose.
“I learned how to talk to subject matter experts that are not data scientists, how to formally present my research and speak to people outside my field,” Turfah said. “I also appreciated being exposed to people at different stages in their academic and professional development—Ph.D. students, postdocs, research assistants.”
Such thoughtful mentorship of the next generation of researchers is only surpassed by Weng’s ongoing work to elucidate study limitations and issues in research design. “Awareness of bias in research has increased in the past few years,” she said. “Those of us working in biomedical informatics feel that it is our role to address this.”
— Karina Alexanyan, Ph.D.