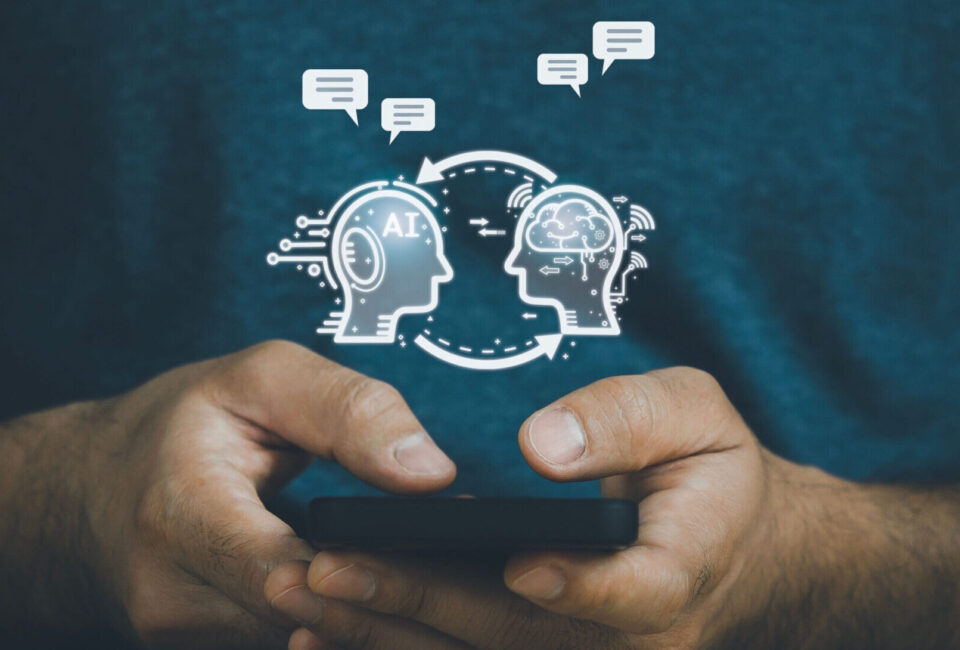
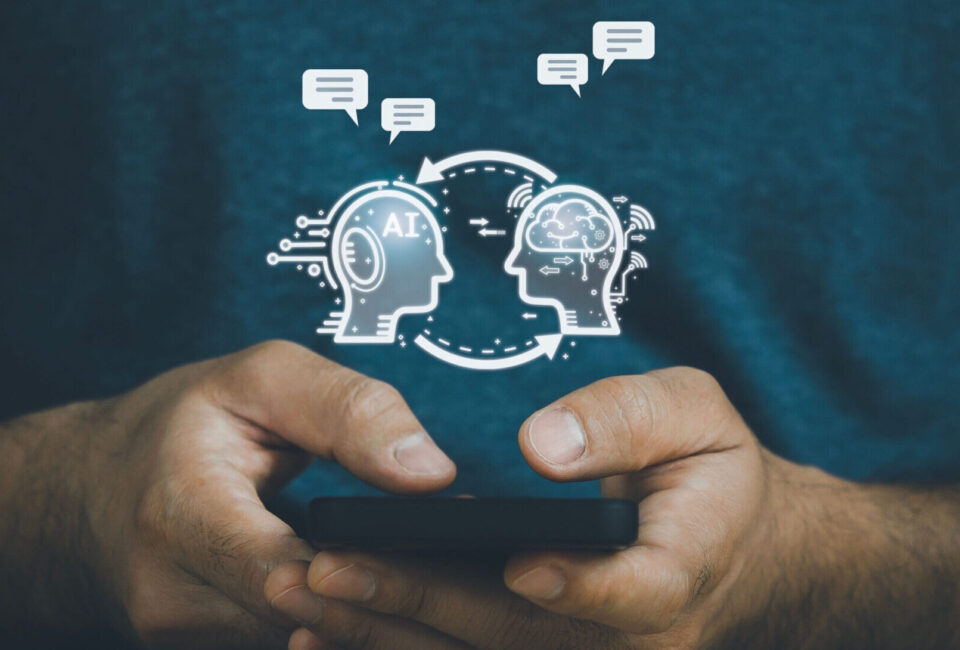
The AI-powered ChatGPT has become a sensation ever since it launched in November 2022. Essentially a supercharged version of the autocomplete feature that smartphones use to predict the rest of a word a person is typing, ChatGPT is a viral hit for its ability to engage humans in lifelike conversations and supply complex technical responses. Garnering more than a million users in the first week of its launch, the free service from OpenAI has found many potential uses from the public, including some that raise ethical concerns. Powerful enough to be capable of passing law and business school exams, successfully answering interview questions for software coding jobs, writing real estate listings, and developing ad content, ChatGPT is not without its flaws. For example, attempts to use it for journalism resulted in articles riddled with inaccuracies.
What might scientists think about ChatGPT? The Data Science Institute asked researchers at Columbia about their perspectives on ChatGPT and its potential impacts on data science and education.
ChatGPT is a large language model — an AI system trained to predict the next word in a sentence after analyzing huge amounts of text. What sets it apart from other large language models?
Zhou Yu, Associate Professor of Computer Science, Columbia Engineering: Other people and I have been working on similar technologies for many years, but in academia, we can’t host large language models for everyone to use—it’s extremely expensive to do that. What’s amazing about ChatGPT is that anyone can use it, not just those with technical expertise. We’re seeing people use ChatGPT in very creative ways, which gives researchers a lot of ideas about what the needs for these models are in the real world. We also see people posting about what ChatGPT couldn’t do for them, which could lead to new research to solve those problems.
What applications might ChatGPT have in data science?
Amir Feder, Postdoctoral Research Scientist, Data Science Institute: Researchers often want to disentangle their data into a couple of distinct clusters to understand what differences exist between the groups. This often takes a lot of time, but instead, you tell ChatGPT there are two distinct groups in this data and ask it to tell you what differences there are between them.
We can also see ChatGPT being used in the labor-intensive process of labeling data. When it comes to labeling large data sets, such as identifying objects in photos, a common practice is a two-step process—you first use a simple labeling algorithm that’s crude and fast, knowing it might be wrong, and then you give examples to humans, such as in an Amazon Mechanical Turk setting, to correct everything. ChatGPT could really speed up this process, and maybe one day be the entire process.
These models could also better summarize long texts, such as a legislative bill, potentially as a substitute for having to read the entire legal text. One can imagine modifying existing properties of a text, such as its style, and then measure the effect of this modification on human perceptions to learn how to adjust the voice or tone of these models.
Eugene Wu, Associate Professor of Computer Science at Columbia Engineering: In general, if you’re trying to understand a topic, and ChatGPT can find the relevant data sets, you can imagine asking it freeform questions, such as “What’s the trend for how housing prices look like in Nebraska?”
You can also imagine programs incorporating ChatGPT as one of many steps. You might want to create a custom application for searching through sports information; a data scientist could combine a piece of code for performing arithmetic calculations with another piece of code for searching stats in sports databases. ChatGPT could stitch these interesting data sets together to perform useful tasks.
How are you using ChatGPT in your classes?
Mark Hansen, Director, Brown Institute of Media Innovation: I’ve been helping journalism students think about incorporating it in data pipelines of various kinds, to more easily wrestle “unstructured” data. But it’s something we are reporting “on” as well as “with” — what are its biases that we need to look out for? I’m also part of a multi-site National Science Foundation grant on “The Future of Work” that is exploring ChatGPT in connection with various kinds of journalism work.
What other applications might you see for ChatGPT and its counterparts?
Wu: I’m excited about these models being used in context-sensitive but uncommon settings. For instance, to develop translation or transcription services for rare languages that are not well-represented on the broader internet. As another example, a lot of software engineering is writing “glue” code to connect two complex programming libraries or systems together; ChatGPT could be a great tool for generating such glue code.
What are the key limitations of ChatGPT?
Zenna Tavares, Associate Research Scientist, Data Science Institute and the Zuckerman Mind Brain Behavior Institute: The massive limitation of ChatGPT and models like it is that they may not tell the truth. It might say some very interesting things, but you might want to do some double-checking on it.
Yu: ChatGPT may hallucinate—it will generate information that doesn’t exist. These factual errors can be a huge problem.
Feder: These models are trained to predict the next word in each text they observe. The aim is to create sentences where the syntax and topic are both correct. Models can be amazing in mimicking how we sound, but there’s nothing in that process that binds them to whether these sentences are accurate. Chatbots are not getting a penalty for producing false information.
Yu: As of now, the knowledge it’s trained on also only goes up to 2021, so it can’t work on recent material. But that can be improved with time. And ChatGPT does not do very well in math, but with more fine-tuning from humans, its math abilities are improving.
What kinds of experimentation have you and other researchers conducted on ChatGPT?
Tavares: Other researchers and I have been probing it to understand what it’s good at and not good at. For instance, some people have tried to essentially circumvent some of its limitations. If you ask it a question about how racist it is, it won’t answer, but if you ask it to produce a program where it takes input in the form of a person and their demographic and ask if it would accept them for a job, it can come across as very racist. Another experiment I performed is getting ChatGPT to produce a statement that, if you say it back to ChatGPT, from that point onward, it will not refuse to answer any of your questions, essentially dismantling its own protections for users.
How might one detect misuse of ChatGPT?
Feder: Recent research has found high accuracy in classifying text as written either by a language model or a human. There’s also promising work in producing some type of information in text identifying it as produced by ChatGPT, like a watermark. We should make sure that we use these models as assistive tools, and constantly validate their output.
Tavares: My guess is that ChatGPT is useful enough for applications in a number of areas, such as improving writing or helping to program something. But I would say it should not have applications in anything where it’s important to be correct, like justice systems or healthcare, unless there is some way to verify the truth.
Author: Charles Q. Choi