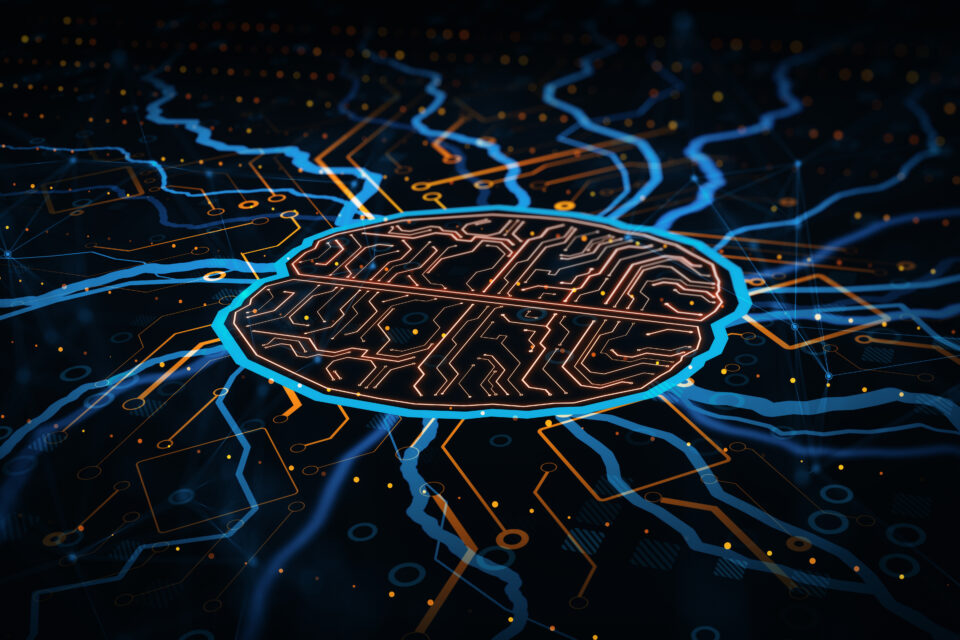
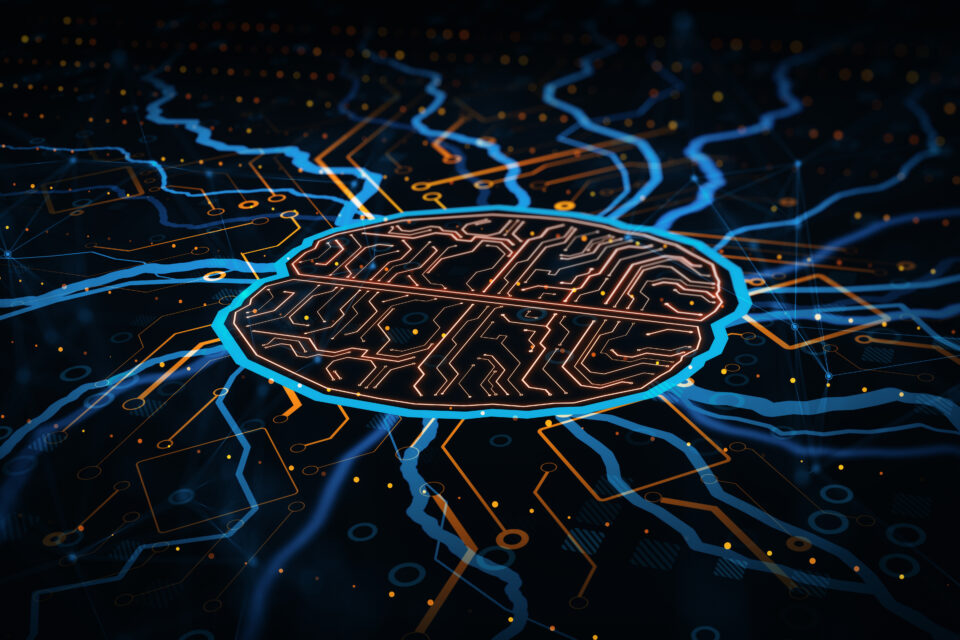
Wei Tang, a Postdoctoral Research Scientist at the Data Science Institute, is focused on building online systems that better integrate human behavior into design and development. Working alongside advisor Shipra Agrawal, his work brings together behavioral and algorithmic economics and interaction design to study how online systems impact our daily lives: from product recommendation systems to internet advertising, revenue management, and resource allocation.
“My work looks at the role of humans in algorithmic design and outcomes,” Tang says. “Specifically – how people influence algorithm design, how human psychology and behavior affects their interactions with algorithms, and how algorithmic decisions affect people in turn.”
This full-circle effect that Tang describes is core to his research process. “My models are built based on a combination of common sense and intuition,” Tang explains. “Then I run behavioral experiments to validate the model.” Leveraging systems like Amazon Mechanical Turk, a crowdsourcing platform that uses workers to support metadata tagging that computers are unable to do, Tang fuses human instinct into each step.
Tang’s recent work looks at information design in human-in-the-loop systems: systems where one side makes decisions about what information to disclose based on a desire to influence the other. Examples include recommendation systems that leverage user reviews, online retailers who vary the presentation of product features in order to influence purchase decisions, or the decisions that public health officials make about how to present public health information (such as data on infection rates or vaccines).
Tang’s most recent research moves away from the standard economic concept that human agents are fully rational towards one where agents may make mistakes, known as “boundedly rational.” He is focused on designing information systems that use signaling schemes which incorporate uncertainty about the agent’s rationality level. This work builds on earlier online behavioral experiments by Tang and his colleagues which found that humans’ online decision-making is not entirely rational.
Tang’s upcoming research involves designing learning algorithms that can help achieve a specific desired outcome and investigating the impact of different policies in dynamic systems. Currently, he is exploring how algorithmic decisions can impact revenue, specifically, how the dynamic pricing of online products affects people’s purchase behavior. “Usually in dynamic pricing, prices can vary across different social groups,” Tang explains. “The question we are asking is – what if people can see prices for other people? How will this affect people’s perceptions of whether they are benefited or discriminated against, and how does this perception affect their purchasing decisions? For example, in location-based pricing – how does setting different prices between regions affect overall revenue?” Tang’s work involves developing a mathematical model and learning algorithms around these dynamics. “Our starting point is a mathematical model to frame the question,” Tang explains. “Then we create theories about optimal pricing and consider how dynamic pricing will affect overall revenue.”
Tang is optimistic about the opportunities presented by DSI and at Columbia. “I appreciate how easy it is to find experts in social science, psychology, and more in New York City, and Dr. Agrawal was someone I really wanted to work with. There are lots of activities, lots of intellectual stimuli – it’s all very exciting.”