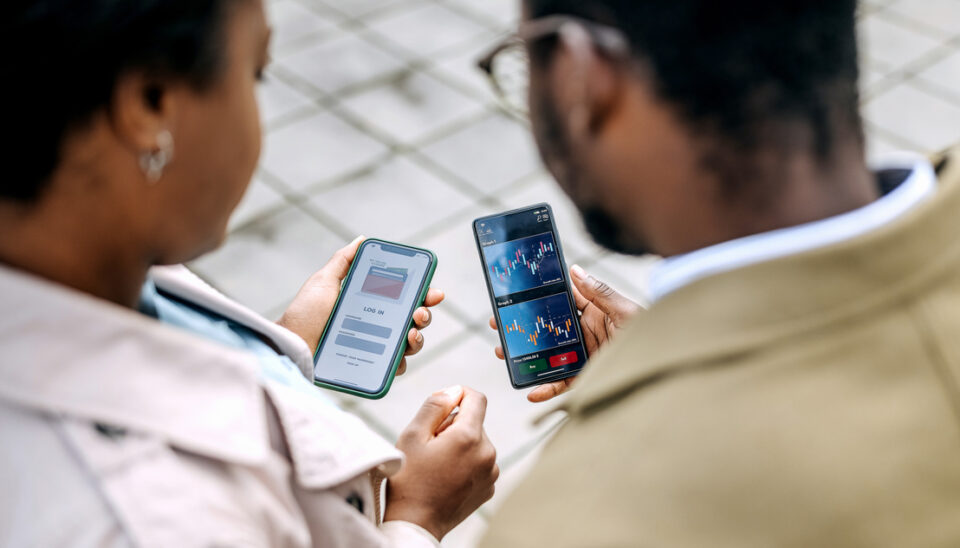
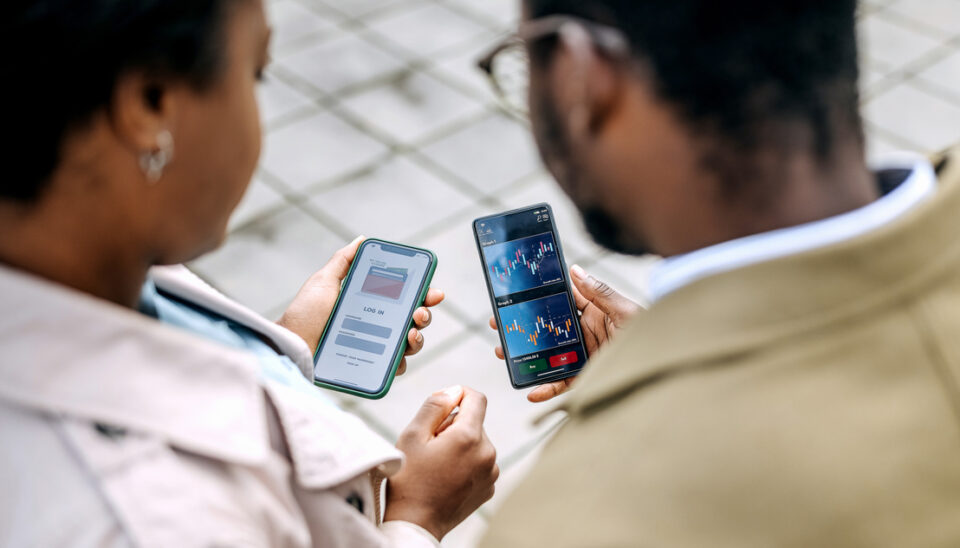
Even as it becomes more common, algorithm-driven pricing faces mounting scrutiny. From restaurants adopting dynamic pricing to allegations against RealPage for inflating rents with nonpublic data, these tools spark fairness debates as well as legislative action: San Francisco and Philadelphia recently passed laws to regulate rent algorithms and protect tenants.
Controversy also extends to entertainment. Ticketmaster’s dynamic pricing recently sparked a UK investigation into unfair practices when prices of an Oasis reunion tour surged more than fourfold.
Adam Elmachtoub is Associate Professor of Industrial Engineering and Operations Research at Columbia Engineering and a member of Columbia’s Data Science Institute who examines how pricing algorithms shape markets and impact society. The creativity and excellence of his research recently earned Adam a Donald P. Gaver, Jr. Early Career Award from the Informs Society, the largest professional association for the decision and data sciences.

Elmachtoub’s research seeks to chart a path forward, balancing innovation with fairness in an increasingly algorithm-driven world. We recently spoke with Adam to help us better understand pricing algorithms and the questions they raise.
Can you explain what pricing algorithms are and where they appear in our daily lives?
Pricing algorithms analyze user data and interactions with products or services to determine prices that optimize specific objectives, such as maximizing profit, improving customer satisfaction, or striking a balance between the two.
These algorithms are everywhere. Ride-sharing platforms adjust fares based on demand and supply. Airlines use dynamic pricing. Even universities use personalized pricing through financial aid, scholarships, and fellowships.
You’ve said there’s no such thing as perfect fairness in pricing. Can you elaborate?
Fairness is subjective and depends on what you’re measuring. Two common types of fairness we study are “price fairness” and “access fairness.”
Price fairness involves ensuring similar prices for the same product across different groups, like Apple and Android users. Access fairness focuses on affordability—ensuring both groups can afford the product at similar rates.
Take universities as an example. Perfect price fairness would mean everyone pays the same tuition, which could exclude low-income students who cannot afford it. Conversely, perfect access and price fairness together would make tuition free, bankrupting universities. The key is finding a balance that works for as many people as possible without creating unsustainable trade-offs.
What are some unintended consequences of trying to embed fairness into pricing algorithms?
Forcing strict fairness can backfire. Consider loans: if regulators require banks to offer the same interest rates to two neighborhoods, banks might stop lending to higher-risk areas entirely, cutting off access to credit.
In ride-sharing, perfect access fairness—ensuring equal access to rides at the same rate—could lead to shutting down the service. If no one gets a ride, it’s technically fair, but it’s also a disaster.
Our research shows that narrowing fairness gaps, rather than enforcing perfection, leads to better outcomes for consumers and businesses. Balancing fairness—rather than overcorrecting—can improve social welfare without crippling the system.
What role does academia play in investigating these issues?
Academia is essential because it operates more independently of profit-driven motives, allowing for a focus on societal impacts. These are complex algorithmic and ethical challenges requiring input from multiple disciplines — engineering, law, operations research, public policy, economics, and more. In addition, businesses often lack the tools or incentives to self-regulate.
This is why interdisciplinary hubs like the Data Science Institute are particularly important. They bring together researchers across fields, and help us provide the space for collaboration, addressing technical, legal, and economic dimensions. For example, at Columbia, I am in regular touch with a legal scholar, Professor Talia Gillis, who is also a Data Science Institute member and we have shared the stage at DSI events.

What got you interested in this field?
My focus is on protecting consumers and building trust in systems. People get stressed about dynamic pricing, whether it’s buying a plane ticket or booking a hotel. One area of my research looks at simple pricing policies, like keeping prices static instead of constantly changing them. We’ve shown that, in some cases, static pricing doesn’t hurt profits and reduces stress for consumers. Transparency and simplicity build trust, and they make maintaining algorithms easier for companies to manage over time.
My work combines fairness and decision-making. Pricing isn’t just a prediction—it’s a decision. Early research on fairness focused on predictions, like credit scores. We’re bringing fairness into decision-making, which is a different challenge.
What’s next for your research?
I’m pursuing two main directions. First, I’m interested in blending machine learning fairness with decision-making fairness. Everything comes from data, so we need to consider both the fairness of predictions (like demand forecasts) and the fairness of decisions based on those predictions (like pricing).
Second, I want to work more with industries and policymakers to bring these ideas into practice. Collaborating with legal scholars, regulators, and businesses is critical to translating research into real-world impact. That’s the long-term goal.