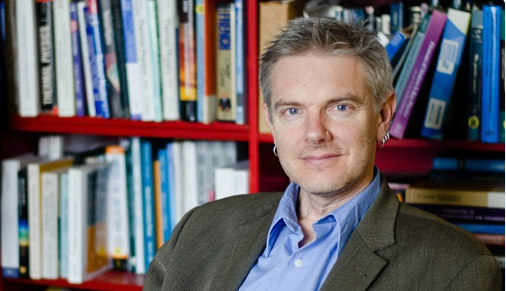
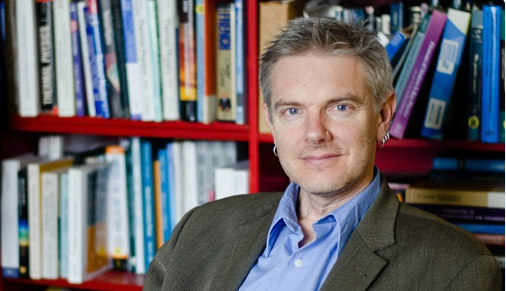
Materials science is vital to solving a wide range of societal problems. An example is a battery that has a high energy density but doesn’t catch fire, and which could someday match the performance of a gas-powered car. And yet, it has traditionally taken up to 20 years and billions of dollars to go from the discovery of a novel material to deploying it in a device.
This lengthy pipeline has created many challenges for researchers – but with new advancements in data science, AI, and machine learning, there are also many new opportunities to speed up this process.
More than 50 senior leaders in materials science gathered for a recent two-day workshop to consider how to tap into these resources and build the materials science lab of the future.
Co-Chaired by Simon Billinge (Professor of Materials Science and Applied Physics and Applied Mathematics), this workshop was part of a series of three recent gatherings sponsored by the National Science Foundation (NSF). While Billinge’s session focused specifically on materials with long-range order (MLRO) – such as bulk crystals, epitaxial films, 2-dimensional materials, and Van der Waals solids – other workshops covered findings in soft and amorphous/solid materials.
The workshop series was developed in response to the updated strategic plan laid out by the Materials Genome Initiative (MGI) in 2021, which aims to better utilize mathematics and data analytics to discover, manufacture, and deploy advanced materials “twice as fast and at a fraction of the cost compared to traditional methods.”
Attendees of the MLRO workshop discussed many of the objectives outlined by MGI, including the policies, resources, and infrastructures the US can build to ensure it remains a global leader in materials discovery. Extending beyond science, participants were also keen to highlight specific needs around human resources, such as technical and automation training for next generation scientists, and forming a more diverse and inclusive space for materials research in educational institutions.
Billinge spoke with DSI about the MGI, key findings from the MLRO workshop, and what they mean for the future of the field.
How is the MGI mission panning out in materials research?
It’s been viewed as a success, but I would say that it’s mostly a success on the theory side. There have been some big developments where people have predicted materials in silico, in computers, and have used machine learning and other approaches (to make such predictions). Some of the predictions were actualized, but it has yet to accelerate things too much.
In 2021, the MGI came up with a new strategic plan for the next ten years. There’s a realization that we need this to cross over into the experiment side of things a bit better. There’s this idea of making a loop of prediction, synthesis, and characterization. They had that vision from the beginning, but there wasn’t really a clear way of figuring out how to do that. That’s still the major challenge.
As the NSF funds lab equipment, they like to hear from the materials community. If we think ahead a little bit to ten years from now, what would our hopes for a lab of the future look like to accomplish the goals of the MGI? How can we develop new materials twice as fast or twice as cheap, or ask scientific questions that are not currently possible to answer?
How does AI impact materials science?
People in materials science are starting to do autonomous experiments. Generally, a student loads a sample, sits down, starts the computer, and the machine does its thing. Then it finishes, and it gives the result back to the student. We’d like to turn this into a closed-loop cycle, where instead of a student sitting there controlling the machine, it’s the computers controlling the machine. Then the computer decides on the next experiment based on the results of the previous experiment, and that’s what we call autonomy. This frees up the student from tedious repetitive tasks for more creative activities. The first paper that demonstrated that experimentally in material science was in 2016.
Will instruments have to be re-designed for autonomous experimentation, or is it a question of how instruments are integrated with AI and each other?
I think that’s what we wanted to figure out. The main finding from the MLRO Workshop was that it’s still a research-grade problem because we don’t know the answer to that question. The equipment is going to kind of look the same, but once you have this completely different modality of deploying the equipment, you can start imagining completely different ways of doing experiments.
I can give a concrete example: we learned how to shoot X-rays in normal incidence (perpendicular) to a thin film to produce an X-ray pattern that we can solve. We’re really good at this now. Once you can do normal incidence measurements on thin films like this, you can develop a “lab on a chip” experiment that is completely different from before.
My group recently collaborated with Tom Mallouk at the University of Pennsylvania, who has figured out how to make a “lab on a chip” using an inkjet printer to print nanoparticle inks onto a surface. The ink has suspended nanoparticles in it that might be useful for catalysis. I can move my beam from point to point and collect data. If I have a 200 x 200 array of inkjet printed spots, I have 40,000 little experiments that I can then probe in one experimental campaign taking no more than a few hours. We can explore this compositional space to find the best catalyst instead of a researcher having to make 20, 50, or 100 samples by grinding powders and mixing them in a beaker. You can just print them like this with slightly different compositions and then measure them.
If we can put it under machine control, it can make smart choices. You can explore a much larger parameter space because the computer has much more patience than humans for doing dull, repetitive tasks, and has the patience to keep making decisions about where to look next.
Will AI take over the materials science lab?
That was definitely another main finding of the workshop, that computers aren’t going to replace scientists. Computers do repetitive stuff really well, and they’re very good at doing optimizations. They’re much more imaginative when doing optimizations. We (humans) tend to vary things a tiny bit. We don’t explore broadly. The things that humans are really good at are making use of intuition and prior knowledge, nonlinear creative thinking, and things that are outside of the prescribed set volume of the parameter space.
It's going to be a partnership between machines and humans. One of the massive challenges that we're going to face is how to make that partnership work: How to have people talk effectively to the machines, and vice versa.
– Simon BillingeWhat other messages came out of the workshop?
Another very interesting possibility is in education and increasing diversity of the STEM workforce. There are some fascinating opportunities where these autonomated experiments could be controlled remotely. You could have a more or less centralized lab, which anyone from anywhere, even high school students, could use to run experiments. You can begin to imagine real citizen science. People would no longer be dealing with toxic materials because a robot is handling the materials – all the while being told what to do by scientists remotely.
In addition to Billinge, the MLRO workshop was co-chaired by Susanne Stemmer, Professor of Materials Science, UC Santa Barbara; and John Mitchell, Senior Scientist and Associate Director of the Materials Science Division, Argonne National Laboratory
Contributing Writer: Jim Kling