Data Science Day 2022
Wednesday, April 6, 2022
5:30 am - 1:30 pm
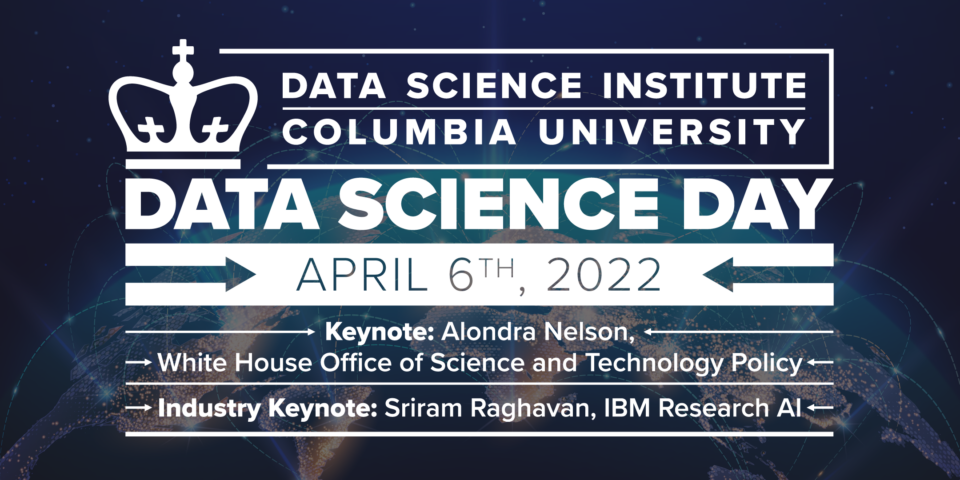
Wednesday, April 6, 2022
5:30 am - 1:30 pm
Data Science Day provides a forum for innovators in academia, industry, and government to connect. The April 6, 2022 hybrid event included two keynote addresses— Alondra Nelson, White House Office of Science and Technology Policy and Sriram Raghavan, IBM Research AI—a series of faculty-led lightning talks, and an in-person poster session on Columbia’s Morningside campus.
All speakers and their respected roles/titles are accurate to time of the event (2022)
Dr. Alondra Nelson is performing the duties of the Director of the White House Office of Science and Technology Policy (OSTP)
Dr. Alondra Nelson is performing the duties of the Director of the White House Office of Science and Technology Policy (OSTP). Nelson assumed this role on February 17, 2022. She leads OSTP’s six policy divisions in their work to advance critical Administration priorities including groundbreaking clean energy investments; a people’s Bill of Rights for automated technologies; a national strategy for STEM equity; appointment of the nation’s Chief Technology Officer; data-driven guidance for implementing the Bipartisan Infrastructure Law; a transformative, life-saving Community Connected Health initiative; and programs to ensure the U.S. remains a magnet for the world’s top innovators and scientists.
Dr. Nelson, a Deputy Assistant to the President, has served since Day 1 of the Biden-Harris Administration as Deputy Director of the newly-created OSTP Science and Society Division. In that role, Nelson directed priority efforts to protect the integrity of science in the federal government, broaden participation in STEM fields, strengthen the U.S. research infrastructure, and ensure that all Americans have equitable access to the benefits of new and emerging technologies and scientific innovation.
She has played a key role in overseeing the implementation of the President’s early directives on Restoring Trust in Government Through Scientific Integrity and Evidence-Based Policymaking and on Advancing Racial Equity and Support for Underserved Communities Through the Federal Government.
A renowned scholar of science, technology, medicine, and social inequality, Nelson has served since 2019 as the Harold F. Linder Professor at the Institute for Advanced Study in Princeton, New Jersey and was previously Dean of Social Science at Columbia University. From 2014 to 2017, she led the Social Science Research Council as the international research organization’s president and CEO, directing historic efforts to apply the insights of social science to the work of making technology development more equitable.
Nelson is the author of numerous books and articles. She is a fellow of the American Association for the Advancement of Science and a member of the National Academy of Medicine and the American Academy of Arts and Sciences.
Sriram Raghavan is Vice President at IBM Research for AI (artificial intelligence). In this role, he leads a worldwide team of over 600 research scientists and engineers across all IBM Research locations who are advancing the field of AI and accelerating its applications to the digital transformation of enterprises. Sriram is responsible for establishing and executing a wide-ranging research agenda that spans foundational and applied AI and works with the commercial arms of IBM to integrate research innovations into IBM’s technology and consulting offerings.
Prior to his current role, Sriram was the Director of the IBM Research Lab in India and the CTO for IBM in India/South Asia. Sriram began his career in IBM at the Almaden Research Center in San Jose, California, USA where he led a variety of research efforts at the intersection of natural language processing, data management, business analytics, and distributed systems. Sriram is an alumnus of Stanford University, USA and the Indian Institute of Technology, Chennai, India. He is a recipient of the IBM Corporate Award for his technical accomplishments and a member of the technical advisory board of the Robert Bosch Center for Data Science & AI.
Talia Gillis
Associate Professor of Law, Columbia Law School
Talk Title: Algorithmic Fair Lending Law: Challenges for Regulating AI
Abstract: Developing an adequate regulatory framework for AI decision-making has become a key focus for lawmakers seeking to capture the benefits of AI systems while protecting vulnerable groups. I use the case of fair lending law to consider current leading regulatory approaches to algorithmic discrimination. One approach, input scrutiny, attempts to address discrimination through policing information used as algorithmic input. While this approach follows fair lending’s traditional approach to discrimination, it is ineffective and threatens to create an algorithmic myth of colorblindness. Another dominant approach to address algorithmic discrimination requires humans to retain decision-making authority. This approach is problematic when discriminatory properties of an algorithm are not well defined or when the human-maker introduces bias. This suggests that leading regulatory proposals, such as the European Union’s recently proposed AI regulation, incorrectly continue to apply old law to new methods.
Tian Zheng
Professor of Statistics and Department Chair, Faculty of Arts and Sciences, Columbia University
Talk Title: Toward a Taxonomy of Trust for Probabilistic Machine Learning
Abstract: Probabilistic machine learning increasingly informs critical decisions in all sectors. We need evidence to support that the resulting decisions are well-founded. To aid development of trust in these decisions, we develop a taxonomy delineating where trust in an analysis can break down: (1) in the translation of real-world goals to goals on a particular set of available training data, (2) in the translation of abstract goals on the training data to a concrete mathematical problem, (3) in the use of an algorithm to solve the stated mathematical problem, and (4) in the use of a particular code implementation of the chosen algorithm. Our taxonomy highlights steps where existing research work on trust tends to concentrate and also steps where establishing trust is particularly challenging. In this talk, I will detail how trust can fail at each step and illustrate our taxonomy with a case study.
Jason S. Adelman
MD, Chief Patient Safety Officer, Columbia University Irving Medical Center/NewYork-Presbyterian
Talk Title: Oops! I Placed an Order on the Wrong Patient: A Case Example of the Use of an Automated Measure of Medical Errors to Improve Patient Safety
Abstract: The 2011 Institute of Medicine (IOM) report, Health IT and Patient Safety: Building Safer Systems for Better Care, raised awareness of patient safety risks introduced by Health Information Technology (IT). The report also noted a lack of adequate systems for quantifying the magnitude of Health IT safety risks and called for the development of new measures to reliably assess the state of Health IT safety and monitor for improvement. In this lightening talk, Dr. Adelman will discuss the use of Electronic Health Record (EHR) log data to develop the Wrong-Patient Retract-and-Reorder measure, the first Health IT safety measure endorsed by the National Quality Forum (NQF Measure #2723). In addition, Dr. Adelman will review how the Wrong-Patient Retract-and-Reorder measure has been used to examine the epidemiology of orders placed on the wrong-patient across clinical settings, and to demonstrate the effectiveness of several interventions aimed at preventing these errors.
Omar Besbes
Vikram S. Pandit Professor of Business, Decision, Risk, and Operations, Columbia Business School
Talk Title: Data-Driven Decisions: How Big Should Your Data Really Be?
Abstract: We consider two fundamental questions in data-driven decision making: 1) how should a decision-maker construct a mapping from historical data to decisions? 2) how much data is needed to operate “effectively”? We discuss various central applications and associated data structures and present recent results that enable to quantify (robustly) achievable performance across data sizes, small and big. These results yield a fundamental practical insight on the robust value of data: in many applications, a little data can go a long way in optimizing decisions.
Moderator: Ying Wei
Professor of Biostatistics, Mailman School of Public Health, Columbia University
Zenna Tavares
Associate Research Scientist, The Data Science Institute, Columbia University
Talk Title: Causal Probabilistic Programming: Towards Machines That Reason
Abstract: Effective decision making on individual, institutional and societal levels requires that we can reliably predict the effect of interventions, discover latent causes, estimate uncertainty, hypothesize counterfactuals, and generally perform reasoning in complex environments. In this talk, I will present causal probabilistic programming as a unifying theoretical foundation for reasoning-based artificial intelligence, and as an increasingly practical technology for analysis and decision making. These systems consume both data and domain knowledge, and automatically compute the answers to various forms of causal and probabilistic queries, presenting a paradigm-shift from machine learning towards more general and human-like machine reasoning.
Richard Zemel
Trianthe Dakolias Professor of Engineering and Applied Science and Professor of Computer Science, Columbia Engineering
Talk Title: Out-of-Context: Data and Models
Abstract: Learning systems frequently fail at out-of-context (OOC) prediction, the problem of making reliable predictions on uncommon or unusual inputs or subgroups of the training distribution. Developing models capable of strong OOC performance is central to research on domain generalization, robust optimization, and fairness. Several benchmarks for measuring OOC performance have been introduced. I will describe a framework we have proposed that unifies the literature on OOC performance measurement, and demonstrate how auxiliary information can be leveraged to identify candidate sets of OOC examples in existing datasets. A promising formulation for OOC prediction is domain-invariant learning, which aims to learn learning which features are specific to particular domains, or partitions of the data, versus those that are domain-invariant. I will present a domain-invariant learning approach that addresses the common setting where partitions are not provided. Finally, I will discuss important connections between invariant learning and algorithmic fairness, with implications for both OOC and fair prediction problems.
Rachel Cummings
Assistant Professor of Industrial Engineering and Operations Research, Columbia Engineering
Talk Title: Differential Privacy: State of the Art and Challenges
Abstract: Privacy concerns are becoming a major obstacle to using data in the way that we want. It’s often unclear how current regulations should translate into technology, and the changing legal landscape surrounding privacy can cause valuable data to go unused. In this talk, we will explore differential privacy as a tool for providing strong privacy guarantees, while still making use of potentially sensitive data. Differential privacy is a parameterized notion of database privacy that gives a mathematically rigorous worst-case bound on the maximum amount of information that can be learned about an individual’s data from the output of a computation. In the past decade, the privacy community has developed algorithms that satisfy this privacy guarantee and allow for accurate data analysis in a wide variety of computational settings, including machine learning, optimization, statistics, and economics. This talk will first give an introduction to differential privacy, and then survey recent advances and future challenges in the field of differential privacy.
Moderator: Ivan Corwin
Professor of Mathematics, Faculty of Arts and Sciences, Columbia University
Data Science Day 2022 included an in-person poster session with more than 60 research posters. The session highlighted new and ongoing work in data science, engineering, and technology underway from Columbia’s faculty, student, and researcher community. Held at Ancell Plaza on Columbia’s morning side campus, this gathering marked a return to in-person event activities at The Data Science Institute.
DSI Industry Affiliates have exclusive access to Data Science Day videos after the event. If you are a current DSI Industry Affiliate, please log into our exclusive website here.
Data Science Day is made possible by the support of the DSI Industry Affiliates Program.